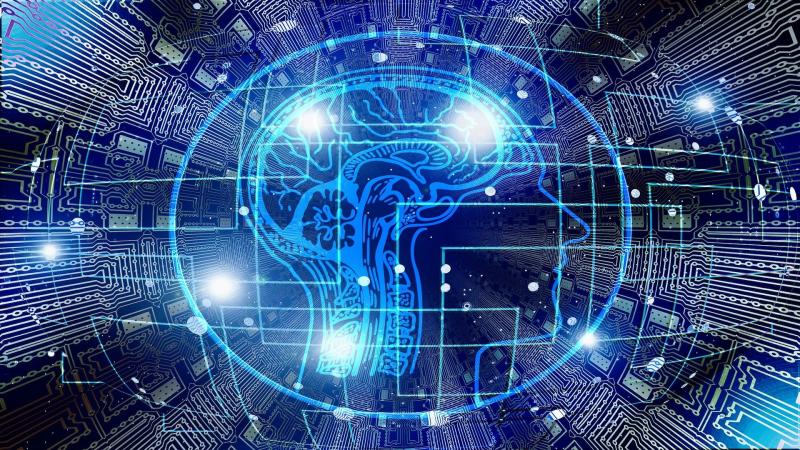
Researchers develop a powerful stochastic neuron, like those in our brain, using random access memory to aid breakthroughs in artificial intelligence
In 2013, Amazon, the world’s biggest online retailer, announced its Amazon PrimeAir service, where drones, flying to your doorstep, would deliver your package in under 30 minutes of ordering. Fascinating? If reports are true, this service could be only a few months away. Advances in machine learning technologies have made innovations like automated drones, which need no human intervention, a reality. While software engineers are coding artificial intelligence into computer programs, building the ‘brain’ behind such technologies, hardware engineers are revolutionising the silicon chips on which these robust programs run.
An active field of research in machine learning is neural networks — a set of algorithms that work like the neurons in our brain and recognise patterns in data. While these algorithms are powerful, they have some limitations.
"Today, a lot of neural networks are focussed on software that runs on the cloud, which have ample energy to work as they are supported by dedicated server farms. However, when these algorithms are used in building self-driving cars or drones, these neural networks have to work on small, mobile devices and have to be energy efficient. This puts focus on neural network hardware," says Prof Udayan Ganguly from the Indian Institute of Technology Bombay.
In a recent study, Prof. Ganguly, his students, and collaborators from the Intel Processor Architecture Research (PAR) Lab, Bengaluru, have designed one such hardware, a type of Random Access Memory (RAM), for neural networks. The study, published in the journal APL Materials, was funded partly by the DST Nano Mission and Ministry of Electronics and IT (MeitY). The work had contributions from a mix of undergraduate and postgraduate students and faculty at IIT Bombay, as well as R&D engineers at Intel.
Most computer hardware designed in the past few decades have a set of circuits whose outputs are deterministic and digital — either a '0' or a '1'. An example of this could be a logic gate, used in most digital circuits, where if you know the input, the output can be determined accurately. This type of hardware served well with simple programs like counting. However, complex problems, such as searching for an optimal route for a drone, need programs that are stochastic. They need to estimate each possible output with some statistical probability. The hardware, in accordance, also needs to switch from being digital to analog to provide that stochastic ability.
In the current study, the researchers have proposed the design of resistive random access memory (RRAM) to enable stochastic neurons. They have considered a theoretical framework of neural networks called a Boltzmann machine, which consists of a network of such neurons.
“A Boltzmann machine can enable everyday tasks like image, voice and pattern recognition,” says Prof Ganguly. "The stochasticity in a Boltzmann machine results in the ability to statistically estimate the output, which is unnatural for deterministic machines," he explains.
The new RRAM, built using a crystalline manganite (PrxCa1−xMnO3), is called PCMO RRAM or a memristor. It is essentially a memory device, whose state is stored in its resistance. For example, the PCMO RRAM could be a resistor with either a high resistance state or low resistance state. A positive voltage causes the resistance state to switch from high to low. This switching is random, and depends on the voltage provided. What is fascinating here is that the neurons in our brain too work similarly. They fire, or send an impulse probabilistically, based on the potential difference between the cell membrane and the axon or the tail. Such a neuron, designed as a hardware, enables a Boltzmann machine.
The researchers then tested their PCMO RRAM by solving a class of search optimization problems that are thought to be difficult to solve computationally.
“The possible number of solutions for such problems grows very steeply as the size of the problem increases. For example, given ‘n’ number of persons, finding how many social groups of different sizes can exist is a difficult problem,” explains Prof Ganguly.
Conventional computers, which are deterministic in nature, would need to evaluate every possibility to find the best solution. The larger the number of possibilities, the more tedious the search task. In comparison, the Boltzmann machine has all the stochastic neurons connected in parallel. When they exchange information by spiking randomly, they eventually reach a specific steady state spiking pattern that indicates an optimal solution, like finding the ultimate answer without the need for a full search. The specific steady state spiking is the lowest energy state of the network. Hence, the network spiking always finds ways to reach this state. This is akin to water flowing downhill or bubbles floating up, where there is a spontaneous change to reduce energy.
“To harness this process, we can set the interactions of these neurons in a specific manner such that the steady-state is the solution to a specific problem,” says Prof Ganguly.
The study compared the performance of the newly-designed PCMO RRAM with that of a conventional silicon-based hardware, which produces analog and digital signals, in solving the optimisation problem. They found that their hardware design solves the problem with 98% accuracy and needs just one-tenth of the area of conventional semiconductor-based hardware. Its power efficiency was also four times better.
"This implies that a Boltzmann machine chip, based on PCMO RRAM, may be computationally more powerful and energy-efficient," says Prof Ganguly.
The study demonstrates the cutting-edge research work underway in India's institutes. The researchers have also filed for a patent on this work.
"The devices are in the experimental state presently, but the chip design needs to be implemented. Such systems are of great commercial interest and would be interesting for high-tech start ups," says Prof Ganguly, before signing off.
This article has been run past the researchers, whose work is covered, to ensure accuracy.