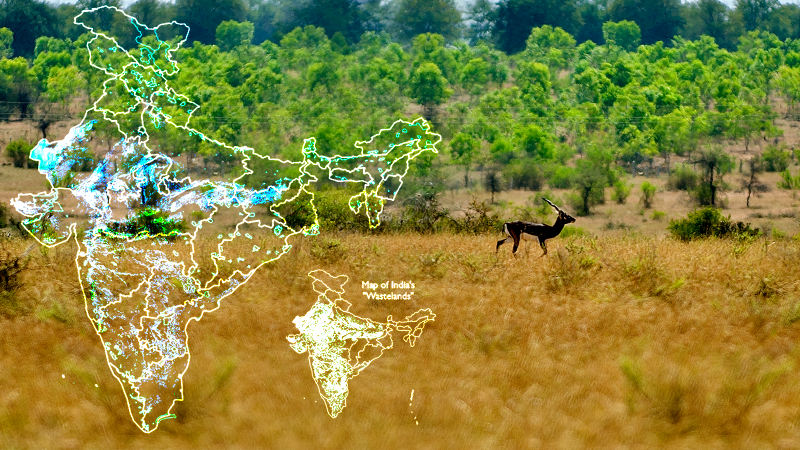
Credits to Graphic: Comparative maps showing the extent of ONEs and wasteland categories in India; Blackbuck - an endemic species of ONEs. Original pictures of Map and Blackbuck - Authors of the paper
There is an urgent need for a policy change to protect India’s Open Natural Ecosystems(ONEs), opine Dr M D Madhusudan, independent researcher and Dr Abi Tamim Vanak, Senior Fellow from the Ashoka Trust for Ecology and the Environment (ATREE), Bengaluru. They argue that contrary to the understanding that these semi-arid, non-forested landforms are barren or wastelands, they are thriving ecosystems. A lack of awareness about their protection is severely threatening the biodiversity of ONEs, endangering species endemic to the regions. During their study of ONEs, they found that the primary challenges lay in the ambiguity in defining, identifying, and mapping the extent of these vulnerable ecosystems. So they embarked upon deriving a high resolution, interactive ONEs map using the Google Earth Engine’s comprehensive earth observation satellite imagery dataset.
Research Matters caught up with Dr Madhusudan to gain insights into their work, currently available as a preprint. Here are excerpts from the interview.
What are Open Natural Ecosystems, and what prompted you to develop a map of them?
We know forests as thriving ecosystems as they have a tree cover and support several species of flora and fauna. On the other hand, diverse, non-forested landscapes such as sandy deserts, grasslands, rocky outcrops, scrub and savannas are landforms that have historically been regarded as wastelands as they have not generated revenue like agricultural or forested lands have done. However, there is more than meets the eye. These diverse landforms are home to unique and rare species such as the great Indian bustards, blackbucks, foxes, fan-throated lizards, to name a few, besides a variety of flora. We label these diverse landforms as Open Natural Ecosystems (ONEs). Moreover, ONEs also support millions of pastoral and agro-pastoral communities who graze their livestock in these areas. Therefore, it would be a very narrow and wholly inaccurate way to think of them as wastelands.
Our research found that ONEs occupy over 300,000 sq km or 10% of India’s landscape, with Rajasthan, Madhya Pradesh, Maharashtra, Andhra Pradesh, and Gujarat having the most prominent patches. We also found that less than 5% of the overall landscape of ONEs come under existing protected areas. Currently, India does not recognise the ecological significance of ONEs, resulting in large tracts of land being categorised as wastelands as they are thought not to offer anything of value to society. Moreover, by representing these as ‘degraded’ or ‘waste’ areas, it ends up looking reasonable to target them for climate change mitigation activities such as establishing renewable energy projects such as solar and wind farms or ‘reforestation’ projects for carbon sequestration. Ironically, such measures pose a further threat and prove to be detrimental to the biodiversity of ONEs. As a result, we are losing ONEs at a far greater pace than rainforests, compromising the survival and habitat of their endemic species.
Earlier work has shown that a lack of awareness in defining these ecosystems, which stems from a bias of forestry classification persistent since the colonial era, severely compromises the habitats. For example, ONEs with woody vegetation are often classified as forests. At the same time, the open landforms are considered degraded lands, with few exceptions, such as the hilly regions of Western Ghats and Himalayas and the Indo-Gangetic and Brahmaputra floodplains.
So to help understand the diversity and spread of ONEs in India, we embarked on generating an extensive country-wide high-resolution map of ONEs occupying the arid and semi-arid regions in India.
The map is powered by Google Earth Engine (GEE). What were the advantages that prompted you to use that platform?
An earlier effort mapped the semi-arid savanna grasslands in India using the medium resolution MODIS imagery. However, at that relatively coarse scale, it was likely that it would be hard to tell apart grasslands from occasionally cultivated or fallow lands.
We found multiple advantages in choosing GEE to develop our map as the platform has curated and archived four decades of earth observation data. First, it offers an up to date, publicly available catalogue of satellite imagery. Second, it also provides value-added thematic layers such as surface water and human settlements. For example, using available datasets on GEE, we could mask out regions that we already knew to be water bodies, thereby narrowing the region within which we needed to identify ONEs that were of interest to us. Third, GEE also provides cloud-based computing infrastructure, advanced algorithms and machine learning options, enabling us to directly process the vast amount of information on the cloud without having to download any data locally. With all these tools at hand, we could generate a relatively fine-scale thematic map over large spatial extents.
What were some of the key aspects for the map that defined ONEs, and what were the training datasets?
We defined ONEs as comprising treeless desert areas including dunes, semi-arid savanna grasslands, savanna woodlands, lateritic plateaus of the northern Western Ghats, rocky outcrops, and other natural treeless open landscapes. In our mapping exercise, we used areas below a 1200 mm annual rainfall cut off and an elevation cut-off of 1000 m. Owing to this, some grasslands, such as those in the alluvial floodplains or the mountain-tops of the Western Ghats, are not included among ONEs that we classified.
Training data are predefined information that helps machine learning algorithms to identify specific aspects, in this case, specific land cover categories. Unfortunately, there was no ready training data available for India’s ONEs. Therefore aggregated training data from various publicly available datasets such as the National Remote Sensing Centre’s Land Use Land Cover (LULC) map and the Wasteland Atlas of India. In all, we aggregated over 180,000 data points corresponding to ONEs and over 110,000 points that were not ONEs. At these training data points, we sampled values from a variety of input bands that represented either vegetation or topographic characteristics. We separated our study area into eight regions. After retaining a hold-out fraction of these data for validation within each region, we developed the model using the training data and tested it on the test-fraction. We combined the output of these models and tested its performance against the validation fraction. Finally, rather than applying our classifier on raw pixel data, we applied this on an input composite whose bands were spatially segmented using a Simple Non-Iterative Clustering algorithm.
How are ONEs distributed across India, and what is the potential expectation from the map?
Our map shows ONEs extend across some 10% of India’s land area, with a heterogeneous distribution; they cover anywhere between 0.4% (Delhi) to 33% (Rajasthan) of the land area in different states. Overall, we observed that 72.7% of ONEs were between 1-10 hectare patches, while 94% were in the 1-100 hectare range; the largest patches (>10000 sq km) were found in the Thar and Kutch regions in Rajasthan. Sadly, only 5% of the overall ONEs are included under the Protected Area network.
Our key aim was to show that ONEs are ecosystems of worth that require urgent policy changes to protect them and to show their locations and extents. We hope that the various land cover categories that constitute India’s ONEs will find their rightful place in vegetation and land cover maps of the country and eventually replace ecologically uninformed and pejorative labels such as ‘wastelands’ and ‘degraded lands.
Hence, we have made the map open to peer and public scrutiny.
[Researchers can analyse, download and export the dataset using this link, while a general user can visualise and use the map using the application].
This article has been run past the researchers, whose work is covered, to ensure accuracy.