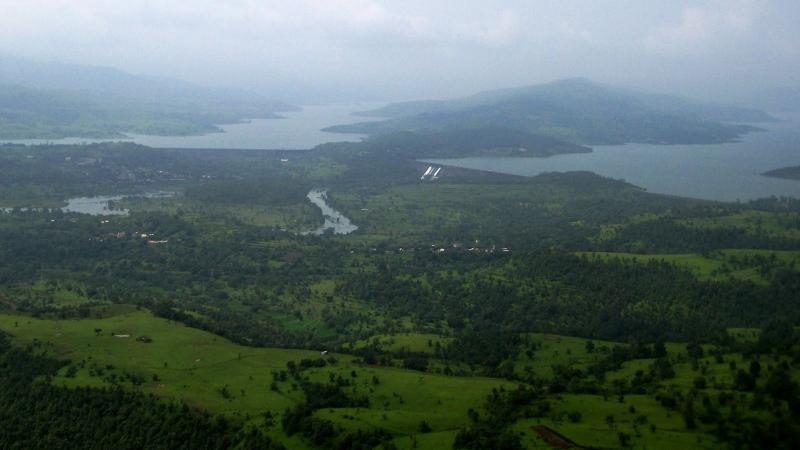
Adding chaos helps finding better solutions to multi-reservoir irrigation systems, says an IIT Bombay study.
Finding the best possible solution to achieve multiple goals simultaneously are of great relevance in our everyday life. Take for example, the irrigation canals in agricultural fields, where it is desirable to ensure that the demand is met for all the areas supplied by the reservoirs. What makes this a complicated problem is that we need to balance both the demand, which depends on the type of crops grown, and the supply that depends on the inflow into the reservoirs. We would like to plan which crops can be grown and in how much area in a given region, depending on the supply capacity and pattern of the reservoir system. In a study, researchers Dr. R. Arunkumar and Prof. V. Jothiprakash of the Indian Institute of Technology Bombay (IITB) have proposed a novel algorithm which can help solve such problems when systems with multiple components are involved.
"Optimizing the operations of a reservoir in itself is complicated because of the uncertainty in the inputs, conflicting and competing objectives, etc. Multi-reservoir systems, with more number of variables to be optimized and large number of physical, technical, legal and social constraints are further complex,” explains Prof. Jothiprakash. To give the best solution taking into consideration all these constraints, multi-objective evolutionary algorithms are the most commonly used.
Multi-objective evolutionary algorithms try to solve problems that satisfy many objectives and are inspired by biological evolution. They start with a initial set of solutions (initial population) which is then allowed to ‘evolve’ under some conditions. Solutions produced after each iteration is tested for optimality. The population is made to ‘evolve’ until an optimal or close to optimal solution set is achieved. If this computation gets stuck at a suboptimal solution, the process will be repeated with a different initial population. As one can see, this is a computationally tedious process and we may not always end up with the best solution.
Here is where the modifications proposed by the researchers of this study come into play. They have suggested the use of chaotic algorithms--algorithms which are very sensitive to changes in initial variables--along with a conventional multi-system evolutionary algorithm that works as above. Studies in the past have shown that using chaotic algorithms have improved solutions with single-objective problems. “Most of the previous studies utilized chaos in only generating the initial population. All those studies reported that the global optimal solutions were obtained more readily and rapidly, when chaos is incorporated,” says Dr. Arunkumar. In this study the chaos algorithm was used not only in generating initial population but also in other steps of evolutionary algorithm.
The researchers also ran simulations using data from Kukadi Irrigation Project (KIP) in Maharashtra, a multi-reservoir system comprising of five reservoirs, to test the efficiency of the modified algorithm. They used the algorithm to find optimal crop plan and water allocation in regions which are dependent on KIP for irrigation. They found that the solutions produced by their algorithm, even though were only slightly better than the conventional algorithms in terms of net benefits and crop area allocation, took far less number of iterations.
“Though there is no significant difference in the optimal solutions obtained from other algorithms, the number of generations taken by the chaotic evolutionary algorithm is much less than conventional algorithms. This shows that the chaotic characteristics may enhance optimization algorithms and make them more successful,” says Dr. Arunkumar about the observed results.
So, can we apply this algorithm to address inefficiencies in our irrigation systems? Not yet, say the researchers. “Real time reservoir operation is more challenging since we will need a system to predict the inflow in real time, along with the reservoir operation model,” says Prof. Jothiprakash. This study is a step in the right direction where we bank on algorithms to help us design public policies and efficient systems.