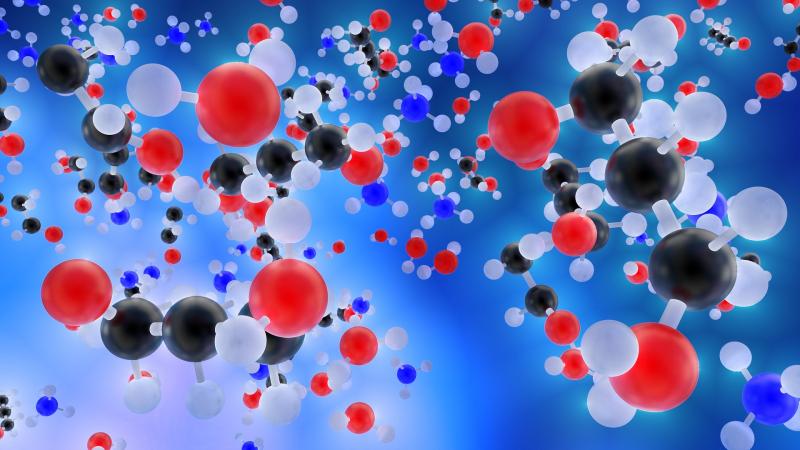
Researchers employ machine learning techniques to search for optimal catalysts for designing pharmaceutically important molecules
Artificial intelligence (AI) and machine-learning (ML) approaches are the present-day buzzwords finding applications in a host of domains affecting our lives. These approaches use known datasets to train and build models that can predict, or sometimes, make decisions about a task. In one such case, researchers at the Indian Institute of Technology Bombay (IIT Bombay), Mumbai, have in a recent study, developed ML approaches using molecular descriptors for certain types of catalysis that could find use in several therapeutic applications.
Traditionally, drug discovery and formulation is an elaborate process. Biological molecules have different properties, the knowledge of which are crucial for binding drug molecules that target proteins. Molecules like proteins and sugars have geometric properties called chirality, where the molecules are not identical to their mirror images. Such mirror images of a chiral molecule are called enantiomers or optical isomers. In other words, an enantiomer is one of the two structures (stereoisomer) whose mirror images are not identical (similar to the difference between our right and left hands).
Building the desired structure of a stereoisomer with the required handedness is a tedious task, typically accomplished using Asymmetric Catalysis. In such an approach, a chiral catalyst directs the formation of a chiral compound such that a particular stereoisomer is rendered more favourable over the other. Knowledge of molecular descriptors (that captures its chemical properties) can accelerate efforts in the discovery of new asymmetric catalysis protocols.
In therapeutic applications, there is an ever-growing need for high-purity chiral compounds that can directly be benefited through the developments in asymmetric catalysis. Often, the creation of new catalysts involves elaborate trial and error cycles and hence is a resource-intensive endeavor. Thus, developing a faster, reliable technique to design such catalysts is necessary.
Conventionally, mathematical models that factor in different considerations for designing such catalysts, including chirality, have been used. This approach involves using many molecular parameters that best fit the regression equation with excellent predictive power. Often this method also poses a challenge to incorporate other nonlinear parameters. Recognising these challenges, researchers at the Indian Institute of Technology Bombay (IIT Bombay) turned towards machine learning to explore if it can aid in their efforts toward identifying asymmetric catalysts.
The researchers have demonstrated a proof-of-concept model to discover better asymmetric catalysts using ML techniques like random forest and decision tree. This approach, the researchers say, can not only accelerate the process but also increase its throughput. In the proposed approach, a ML-based mathematical model is trained on known catalysts, which then helps to predict the effectiveness of other catalysts. After multiple such training runs with additional data of catalysts, the model was validated with some test sets. This process of train-predict-train cycle accelerates the discovery of favourable catalysts.
The predictive power of the random forest built using the molecular parameters of a set of substrate–catalyst combinations were found to be impressive. The accuracy of the random forest was found to be superior to other ML methods. This approach is expected to provide a leap forward in the design of catalysts and could have far-reaching implications in studying and expanding the repertoire of asymmetric catalysts and substrate libraries.
“We believe that the leads emerging through machine learning on what combination of catalysts and substrates has better propensity to be successful and could be coupled with automated experimental protocols. Our approach can also be exploited in a broad range of asymmetric reactions and thus can open up promising avenues toward a cost-effective and efficient design of asymmetric catalysts,” signs off Prof Raghavan B Sunoj (Department of Chemistry), who led this multidisciplinary study along with Prof. P Balamurugan (Industrial Engineering and Operations Research).
This article has been run past the researchers, whose work is covered, to ensure accuracy.