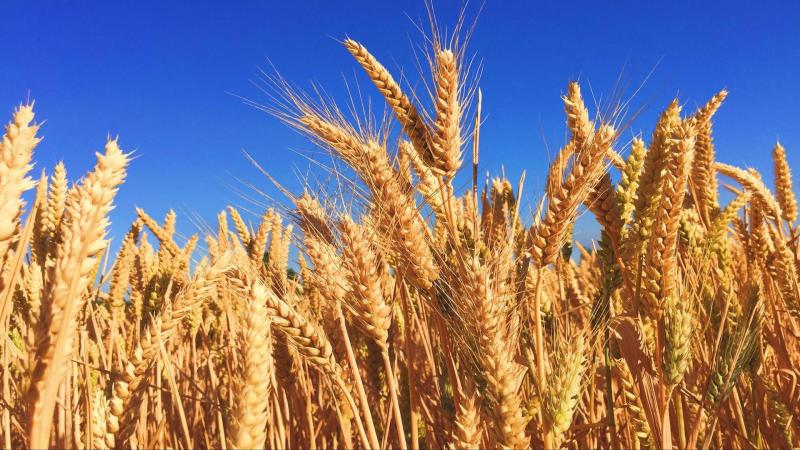
Researchers use radar data from satellites to estimate parameters that determine the growth of soy and wheat.
The eyes of satellites see what we cannot. Google Street View, for example, takes you virtually to a street that could be thousands of miles away and puts you in the centre of a road. It does so by using satellite data that captures every detail of the planet at a very high resolution. Many such remote sensing satellites are used for military applications too. In a recent study, researchers at the Indian Institute of Technology Bombay (IIT Bombay) and Agriculture and Agri-Food Canada have used satellite data to monitor the growth of crops.
In agricultural countries like India, monitoring crop growth is vital for food security and the economy. However, with about 60% of the area under agriculture, physically monitoring is tedious and unviable. Here is where satellites could help us. Data from remote sensing satellites includes radar scans of the Earth’s surface and is widely used for forest mapping and monitoring. Using various biophysical parameters, one can estimate the biomass in a given area.
In the current study, the researchers have estimated three biophysical parameters that determine crop growth of wheat and soybean over Manitoba, Canada, using satellite data. These included the leaf area index, biomass, and plant height. The leaf area index, as the name suggests, is the leaf area exposed to the sky per unit ground surface and determines the foliage and canopy structure. The biomass parameter determines the water content and the amount of carbon accumulated.
The researchers began by constructing a mathematical model to retrieve vegetation information from satellite data and observations. They used a model called Water Cloud Model, which assumes that the water content in the crop canopy is scattered as in a water cloud. This assumption provides an accurate model to analyse the reflected radar signals obtained from satellite data. The model and mathematical relations are constructed using a process called ‘model inversion’, which is then used to determine various plant growth descriptors such as crop height by using radar data as the input.
In the current study, published in the International Journal of Applied Earth Observation and Geoinformation, the researchers have used machine learning to carry out model inversion. Machine learning consists of a set of statistical models and algorithms that enables a computer system to learn from data without explicit instructions. A familiar example of machine learning in action is the set of recommended products that you are shown on an online shopping website based on your search and shopping history.
Machine learning methods typically map several input parameters to determine one output. If multiple outputs are needed, these algorithms have to be run exclusively for each of the outputs. However, when the outputs are non-linearly related, the final result becomes error-prone. Hence, we need an algorithm that does not overlook the relationships of the outputs.
“We have identified that such a relationship among leaf area index and plant biomass needs to be preserved for model inversion, as they are directly related to crop yield,” says Mr Dipankar Mandal who investigated the experiments as a part of his doctoral research work.
Conventional algorithms, like multi-output support vector regression, are memory intensive and do not perform well with large datasets. The researchers of the current study used an algorithm called multi-target random forest regression (MTRFR), which takes into consideration the relationships between the outputs. They used satellite data to estimate the leaf area index, biomass, and plant height for wheat and soybean in the Red River watershed of southern Manitoba. They found that the newly designed model not only replicated the data but also preserved the relationship between the outputs. This approach was more accurate than conventional algorithms.
Although the model was used to estimate crop parameters in Canada, it can be used in India with some changes, say the researchers.
“Unlike Canadian fields, Indian fields are smaller in size and have varied cropping patterns. Hence, we may need high resolution and time-dependent data,” says Dr Avik Bhattacharya from IIT Bombay, who led the study.
Consequently, the inversion strategy needed will also have to change.
As the next step in this research, the researchers plan to use their findings to assess the risk in crop production. They are also finding ways to optimise their algorithm so that they can use it on large scale data.
This article has been run past the researchers, whose work is covered, to ensure accuracy.