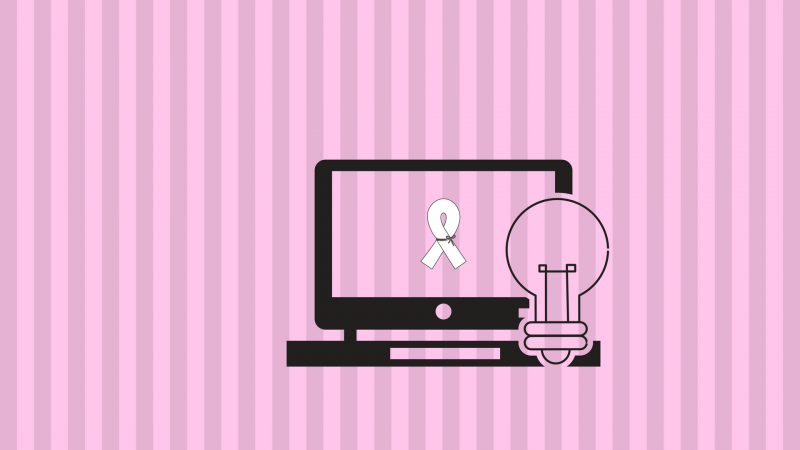
Researchers at IIT-Kharagpur have published a study on prediction of Esophageal cancer using data locally collected by a Mumbai hospital and machine learning algorithm. Their results could help us do away with expensive and invasive tests while diagnosing cancers.
The concept of using computers to help in medical diagnosis isn’t exactly new, but homegrown research in the application of machine learning algorithms for cancer prediction - making a medical practitioner almost redundant at the screening phase - that is not common and is worthy of attention. The new study does just that.
Esophageal cancer affects the food-pipe and is the fourth most common cause of cancer-related deaths in India. Usual diagnostic methods include various kinds of imaging tests using x-rays (Barium swallow/CT scan), magnetic fields (MRI scan), endoscopy (a camera in a tube, passed down the throat), or even a biopsy (a small piece of tissue removed and examined).
The researchers wanted to eliminate these tests as a necessary step in the diagnostic process.They set out with the aim of predicting occurrences of esophageal cancer in suspect cases, with as low a ‘false negative rate’ as possible, using information available from the electronic medical records (EMR) of the patients. A false negative is where a cancer patient is not predicted to have the disease - these need to be minimized to restrict the number of cases that go unpredicted, and hence potentially untreated.
The data used includes demographic details (e.g. age, education, occupation), lifestyle choices (e.g. consumption of tobacco, alcohol) and the patient’s medical history. Four commonly used classification algorithms, Logistic Regression, Support Vector Machine, Random Forest, and Naive-Bayes classifier, were used for the prediction. These algorithms are methods for grouping members of a population (patients, in this case) in different classes (having cancer or not) utilizing some underlying features of the members (EMR data).
The study establishes that one can successfully predict cases of cancer with an overall accuracy of more than 90% while keeping the false negatives at almost zero. Patients can thus ascertain they don’t have esophageal cancer without going through the expensive and invasive tests, which in turn also eases the burden on the healthcare system.