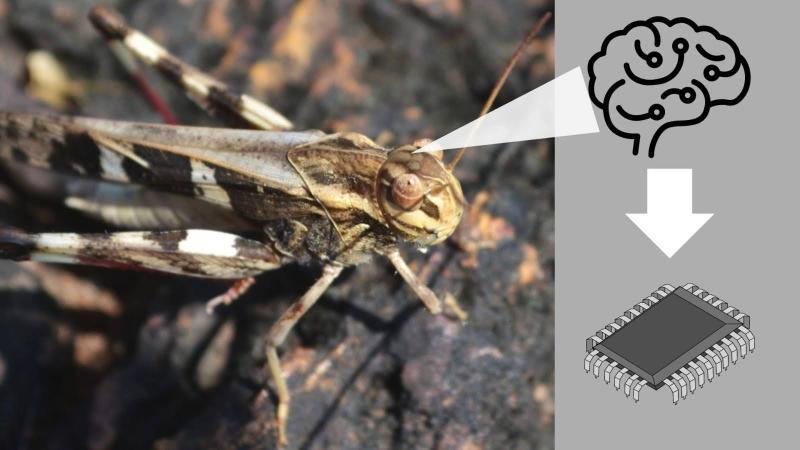
In the age of artificial intelligence (AI), there are many exciting developments from chatbots powered by large-language models to autonomous vehicles and self-driving cars. We are in a very exciting phase of continuously evolving AI, witnessing how these innovations are unfolding and impacting our lives. While Tesla’s self-driving cars have hit the market in many other countries, India’s own Pragyan rover (built by ISRO) navigated itself on the unchartered surface of the moon.
One of the key challenges in autonomous vehicles is the ability to accurately and quickly detect moving obstacles. The existing obstacle detection systems, based on complex algorithms and vision systems, are often inefficient in terms of energy consumption and size. In a recent study, researchers from the Indian Institute of Technology Bombay (IIT Bombay) and King’s College London, United Kingdom have designed and built an ultra-low power transistor, which when incorporated into their artificial neuron circuit design, is capable of obstacle detection. The circuit mimics the spiking neuron model of biological neurons.
The researchers were motivated by the brain's unique ability to process information in a distinctive manner. Particularly, they took note of the behaviour of a collision-detecting neuron found in locusts. The neuron, called lobula giant movement detector (LGMD), plays a crucial role in helping locusts avoid collisions with objects in their path. The mechanism is similar to the way a computer works, but the brain does it in a much more energy-efficient way. In the current study, the team has designed a new type of low-power artificial neuron circuit that closely mimics the behaviour of this collision-detecting neuron found in locusts.
The novel artificial neuron circuit is designed by incorporating the models of a new subthreshold transistor built using a two-dimensional (2D) material channel. The use of ultra-thin 2D materials allows reconfigurable and low-power operation, making it suitable for energy-efficient applications. The transistor was carefully designed and fabricated to replicate sodium channel behaviour in biological neurons besides operating under a low-current regime, which enhances its energy efficiency.
Explaining the rationale behind choosing a 2D material for the transistor, Prof Saurabh Lodha, from the Department of Electrical Engineering, Indian Institute of Technology Bombay (IITB) and lead author behind the study says, ”Unlike modern computers, the human brain consumes extremely low power for memory and computing. Hence, low power consumption is a key requirement for neuromorphic (modelled after the human brain) electronics. 2D materials are ideally suited for this purpose due to their atomically-thin nature that allows for excellent electrostatic control leading to low-power operation. Although conventional semiconductors such as silicon can be thinned down as well, they lose their performance dramatically at scaled thicknesses, unlike 2D materials”.
The researchers demonstrated the low power consumption in simulations by incorporating the model of the newly built transistor into a neuronal circuit. They showed that the artificial neuron circuit closely matches the essential computational features of the LGMD neuron. It can generate LGMD-like spiking behaviour, wherein voltage spikes are produced in response to an input current signal, and detect obstacles at a low energy cost. The energy per spike of the artificial neuron is estimated to be around 3.5 picojoules (pJ), which makes it highly energy efficient compared to existing biomimetic spiking neurons.
Recollecting the challenges the researchers faced, Kartikey Thakar, the first author of the recent study notes that, “The main challenge was to achieve all essential features and spike times to match the biological LGMD neuron response. Another major challenge was the minimisation of the total energy dissipation of the entire circuit to the best-in-class among other 2D material-based reports. Careful design of the 2D subthreshold transistor characteristics played a critical role in achieving both of these results, which helped the work stand out among its peers”.
The LGMD-like neuron circuit, when provided with inputs mimicking collisions, was able to accurately detect looming objects, signalling a potential collision, at an energy cost of less than 100 pJ. Furthermore, the circuit could distinguish between looming and receding objects, providing a selective response to approaching objects in the direct collision path. This selectivity is crucial for prioritising the system's response to potential threats. The artificial neuron also continues to function reliably even when there are variations in the current or noise in the input, making it robust and reliable for real-world applications.
The results of this research have important implications in the field of autonomous robotics and vehicle navigation. The ultra-low power spiking neuron circuit could be seamlessly integrated into existing systems, enabling accurate and energy-efficient obstacle detection. This could greatly enhance the safety and reliability of autonomous vehicles operating in unknown or dynamic environments.
Prof Bipin Rajendran, Department of Engineering, King’s College London, and a co-author of this study, says “We demonstrated that this spiking neuron circuit can be used for obstacle detection. However, the circuit can be used in other neuromorphic (systems mimicking the human brain) applications based on analog or mixed signal technology that require a low-energy spiking neuron.”
Speaking on the prospects of translating this to the market, Prof Saurabh Lodha says that, “the semiconductor industry has shown increasing interest in 2D materials for its future transistor roadmap. Their wider adoption in the industry will greatly depend on the nature of the solutions to some of the technological challenges related to 2D materials-based devices. Specifically, these solutions will have to be compatible with existing technology platforms from a process and complexity perspective, be it logic, memory or MEMS, easily integrable with other features on the semiconductor technology roadmap, e.g. heterogeneous integration, and enable future technologies such as quantum computing”.
Overall, this research represents a significant advancement in the field of neuromorphic engineering and autonomous robotics using 2D materials in the development of low-power spiking neuron circuits. The research findings can potentially revolutionise obstacle detection and avoidance and pave the way for further exploration of advanced neuromorphic systems and their integration into real-world applications.