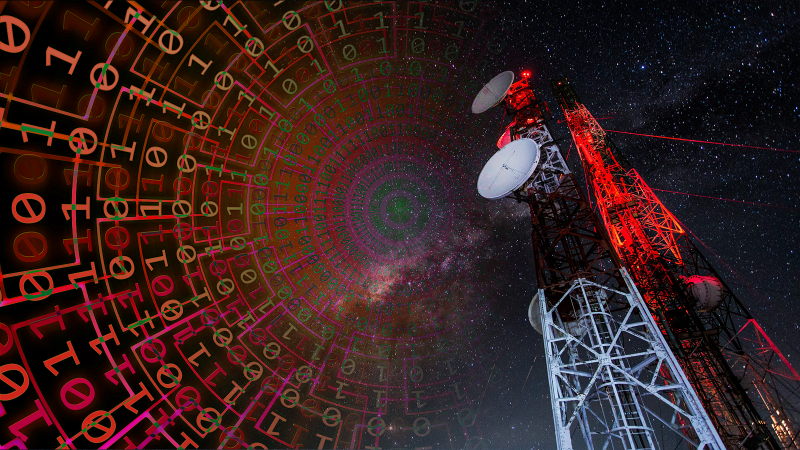
Radio Frequency (RF) signals are electromagnetic radiations used in wireless communication. RF signals transmit information and carry an inherent small electrical energy component. Emerging technology harvests this electrical energy and powers many wireless devices (called nodes) over a wide area, such as medical implants or IoTs.
RF energy is harvested either by scavenging the ambient energy or by having a dedicated energy source. Such a system facilitates the continuous charging of the nodes’ batteries, enhances their life and overcomes the energy limitations of conventional battery-powered wireless devices. Also, it reduces the need for frequent battery replacement, not only from the practical and economic perspective but also makes it easy to use the nodes in hazardous environments. However, RF energy harvesting networks consume high energy for both energy and information transmission. Therefore, optimising energy losses is a crucial and active research area for future wireless communication networks.
Wireless nodes detect, monitor and report the energy harvesting status to the energy source. Based on the feedback, the source regulates the right power level to meet the demand and avoid power wastage. Researchers use statistics-based algorithms driven by artificial intelligence to automate the power optimising process.
Current algorithms are designed on a metric called channel state information: the feedback from the receivers, such as how good the link is or how much of the received energy they could use. In such smart systems, the power sources act as both transmitters and receivers of information. The power source has to assess how much energy has to be transmitted so that all the nodes get enough energy to transmit information. However, more energy to the nodes does not translate to more information transmission. Hence it is important to evaluate the overall energy efficiency of the RF-energy harvesting network.
A team of researchers from the Indian Institute of Technology Bombay (IIT Bombay) and Monash University, Australia, has developed a new algorithm that identifies the right amount of power level, enhancing the energy efficiency of an RF-energy harvesting network. Their algorithm uses a statistical tool called the multi-armed bandit method that does not depend on channel state information parameters, and the source identifies the optimal power output. The performance results of the algorithm are presented in the journal IEEE Wireless Communications Letters.
The project is funded by the Department of Science & Technology (DST) and Science and Engineering Research Board (SERB), Govt of India, through the Innovation in Science Pursuit for Inspired Research (INSPIRE) Faculty Fellowship and Early Career Research Award (ECRA); and the Australian Research Councils Discovery Early Career Researcher Award (DECRA) Scheme.
“An actual transmission system is a complex network with several receivers spread over a region receiving different amounts of energy for harvesting. Also, they will require different amounts of energy for successfully transmitting the information,” says Prof Manjesh Hanawal, lead author of the study.
As the environment is uncertain, reinforcing the algorithms with sequential decision making can quickly ascertain the status of the harvested energy, thereby improving the system’s energy efficiency, he adds. However, traditional optimisation techniques drastically increase computation costs as they require information on the channel state parameters.
To overcome this hurdle, the team used a sequential optimisation method in the algorithm called the Multi-Armed Bandit technique that relies only on detecting if a receiver’s feedback signal was successfully decoded or not (a yes-no status). This technique is akin to exploring multiple levers and playing the best lever of a slot machine (gambling devices) at a given time. First, the player risks a few losses by exploring the levers; then, sequentially pulling the levers, the player learns the lever that maximises the overall reward after some trials.
The source selects a power level in the harvesting network to transmit energy at a given time slot. The nodes harvest this energy, and using this energy, they send back information to the source. If the nodes could harvest enough energy, they will be able to transfer the information higher than a certain rate; otherwise, no information transfer will occur.
“The rate at which nodes could transmit the information is treated as the reward and is directly coupled with the amount of energy harvested,” explains Ms Debamita Ghosh, first author of the study.
In traditional optimisation methods, as the source power increases, the rate of transmission also increases. However, receivers cannot harness the energy indefinitely due to physical limitations, and the rate of information saturates, leading to transmission losses, thereby compromising the network’s energy efficiency.
So, the team considered the rate of information per unit of power, i.e. bits/second/Joules, instead of the rate of information from the nodes. “Since there could be multiple nodes in the network, we consider the total rate of information of all the nodes per unit power as the performance metric,” says Ms Ghosh. The source selects the transmit power in each time slot to transmit energy such that it receives the maximum possible bits at the source per unit of power spent, she adds. Also, the algorithm estimates an upper limit of the mean of the total rate of information per unit power for each power level and uses the power level with the highest estimated bound level.
Thus, though there are few initial losses for not playing the best power level, overall, the accumulated losses are minimised due to the sequential learning. In addition, the team performed simulations of algorithm outputs to establish that it helps the source optimise the power output, thereby improving the system’s energy efficiency compared to current computation methods.
This article has been run past the researchers, whose work is covered, to ensure accuracy.