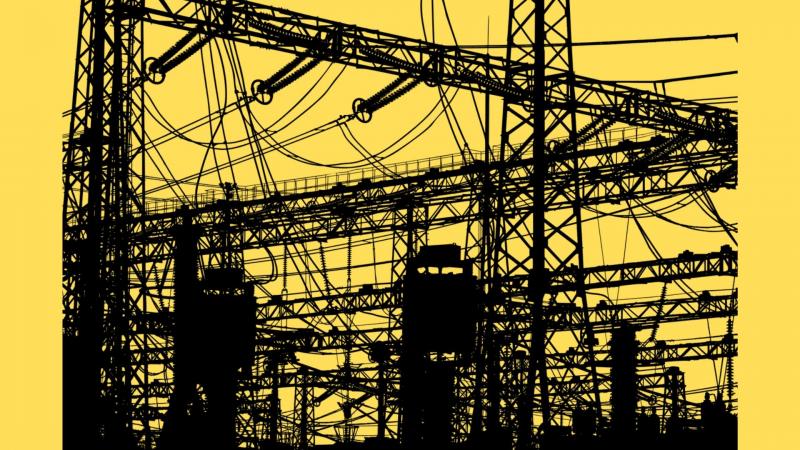
There is a good chance that the electricity that powers your devices at home is generated at a powerplant miles away from you. Take for instance the Vindhyachal power plant in Madhya Pradesh, the largest in India, which supplies power to many states, including Madhya Pradesh, Chattisgarh, Gujarat, Maharashtra, and Goa. Such a design results in a substantial loss of electricity during transmission. Moreover, when a crisis like equipment failures or natural calamities strikes the power plant, it affects all the dependent areas.
An alternative to this design is microgrids—a smaller setup of power generation, consumption, and storage serving a small neighbourhood—which is now gaining popularity. In a recent study, researchers from the Indian Institute of Science (IISc), Bengaluru and IBM Research-India have developed a machine learning-based technique to manage the demand and supply of power in a network of microgrids while maximising profit. Since such local grids can run on renewable sources of energy instead of relying on fossil fuels, they also reduce carbon emissions and are sustainable in the future.
A city could have many such microgrids, which can be connected to the primary power grid or other microgrids to buy and sell power and meet the demand and the supply. The behaviour of the connected microgrids can impact the overall operation of such a network. Most electricity boards are modeling this interplay and studying its impact on the primary grid. However, the sheer number of microgrids on the system and their unpredictability to generate power makes this a challenging task.
One approach to satisfying the demand is to manage energy distribution on the supply side just among the microgrids. This method can significantly reduce the wastage of power and reliance on the primary grid. Alternatively, it is also possible to manage peak demands by load shifting—moving the consumption of load to different times within an hour, a day, or a week. This approach does not reduce the net quantity of energy consumed, but changes when it is consumed. With smart meters that communicate with electricity boards and smart appliances that can be run at a specific time when the peak load is less, it is possible to shift the load.
With microgrids powered with renewables, the amount of generated power can vary based on the availability of water or the intensity of wind to run the turbines. The ultimate goal, however, is to make a profit by selling excess power and ensuring constant supply when there is demand. Hence, managing power generation requires instantaneous decisions to be made, which cannot be done by humans alone.
Hitherto, the grids were coordinated using models that relied on classical control theory where the engineers aimed to model the whole system. “The previously used techniques have become very tedious and highly complex as the number of microgrids in the network increase,” says Prof Shalabh Bhatnagar from IISc, who is an author of the study.
However, it is possible to coordinate with a good approximation of the system. Hence, statistical methods that decide on what action to take based on the available demand/supply data, without bothering about the underlying network, are useful.
A statistical model in this context has variables representing microgrids, whose value is assigned by its state of power exchange. Possible states include receiving power, selling power, or not trading power. The researchers use a machine learning technique called 'reinforcement learning' to search through the states of the microgrids to make the system efficient and profitable. In reinforcement learning, a model learns to make decisions through rewards or penalties it incurs for each action it takes, thus improving itself in the process. The model learns to make better decisions over time without the need to know other details of the entire network.
In the present study, the researchers have integrated both the demand side and supply side management problems of a network of microgrids. Their model takes in information regarding the power generated at each microgrid, the demand from customers, the price of electricity, and the capacity of the battery at each microgrid. It then decides to store, sell, or buy power either from the primary grid or other microgrids.
"A microgrid operating on solar power can sell excess power during the morning as it can generate electricity for the most part of the day. However, it may not be a good choice to aggressively sell it in the evening as there will be no power generation during the night," explain the researchers, citing an example of the dynamics of the model.
The researchers tested their model on two simulated networks with three and five microgrids. Some of these microgrids ran on solar energy and some on wind energy. They observed that the solar microgrids tended to sell excess power, as they generate electricity throughout the day. On the other hand, wind-powered microgrids bought electricity to store when the demand was expected to be higher. The model was also able to increase profits from each microgrid significantly.
As a next step, the researchers are developing a pricing model for such networks, where microgrids can automatically bid for the price of power during trading to maximise their profits. "Once we do that, we will consider deployment in real applications," shares Prof Bhatnagar.