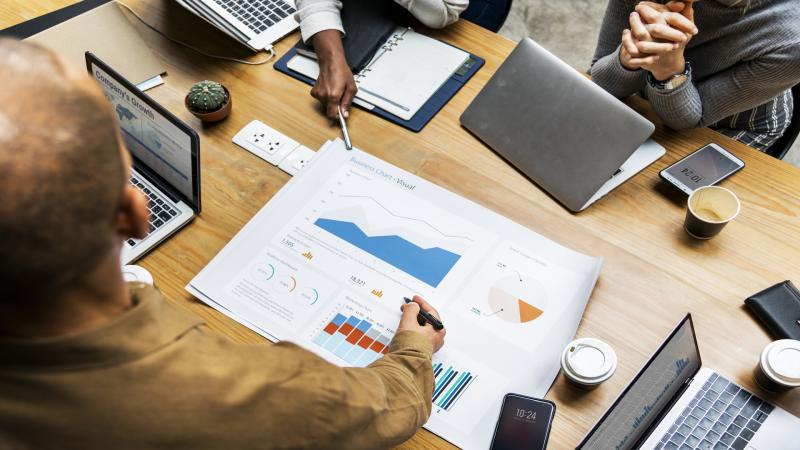
In an earlier episode of The Joy of Science Shambhavi Chidambaram spoke to Professor Shravan Vasishth about, among other things, the joy of psycholinguistics. In this interview, Professor Vasishth talks in detail about teaching statistics and the need to understand uncertainty both to students and the general public. He is the author of “Shravan Vasishth’s Slog”, a blog about statistics. This interview has been edited for clarity and conciseness and has been run past Prof Vasishth for accuracy before publication.
SC (Shambhavi Chidambaram): In today’s world, there is a rapid change in how science is done, partly because of the pace of technological development. From an education perspective, at the Bachelor’s level, Master’s level, and PhD level, what should students be given access to, for a good statistical grounding?
SV (Shravan Vasishth): Access to the right resources, especially in the Indian context, is a very big problem. I studied in India, and we had a complete lack of resources. So what I’m doing right now is adding to freely-available resources systematically. I’m preparing materials that will be available online for free. My books will be available for free. I have a contract with the publisher, that my books will always be online. I’m also preparing video lectures that will be available for free on YouTube, so they can watch everything. Coursera also has free courses. But I think it’s up to this young generation in India to access these free tools. I don’t think they can rely on institutional changes. What will happen is that these young people will learn the new stuff, and will apply it when they become professors. This is already happening: one of my former postdocs, Professor Samar Husain, is a professor in Delhi, and he is really revolutionizing the study of psycholinguistics in India right now.
SC: In your courses that you have made available for free, you cover both Frequentist as well as Bayesian statistics. Many people are only familiar with the Frequentist framework, which draws conclusions from sample data by emphasizing the frequency or proportion of the data. What is Bayesian statistics? Why do you prefer to work with Bayesian statistics than Frequentist statistics?
SV: This is a very important question. For me, the Bayesian approach is the only viable approach for data analysis because its focus is on uncertainty quantification—a key phrase that we need to understand. It’s a very deep phrase and has very deep implications. To give you a day to day example, think about the weather. If somebody says there’s a 60% chance of rain, that’s a point value. As a Bayesian, what I want to know is, what is the range of chance of rain? How sure are you of that 60%? Maybe you’re 95% sure that the chance of rain is between 20 and 80%. That is a lot of uncertainty. If you had instead told me, I am 95% sure that the chance of rain is between 55 and 60%, that is a very tight interval and that tells me that you have a lot of information. For me, the entire action is on how uncertain I am. The Frequentist approach, on the other hand, rejects a completely irrelevant hypothesis. The null hypothesis is not something I actually care about. I care about the alternative. Even if I care about the null, I care about how unsure I am that the effect is real.
Editor’s Note: A null hypothesis is the default assumption in statistics that there is no real difference between two groups. Testing a hypothesis usually involves testing a null hypothesis and either accepting or rejecting it if the difference between the groups is found to be ‘statistically significant’.
Until the 1990s, it was not possible to do serious computational Bayesian analysis. It has only become tractable since 2013 onwards. Now, I think things will change because people realise that they can actually answer the correct question! Since the Frequentist paradigm rejects a null hypothesis, it doesn’t tell you about the specific alternative you’re interested in. As a Bayesian, I can answer the specific question I care about.
SC: The other development we see in recent times is the technology to do science in an open way. It’s now possible to put your raw data and your study design online, and this is an effective way of preventing scooping, because you’ve time-stamped it, you’ve put this online and said ‘I’m working on this now.’
SV: Today, many scientists refuse to release data. In fact, 75% of the people I have asked for data have refused to give it to me. What this means is that they are afraid that they’ll be scooped, or that I’ll find something new in their data or point out a mistake. This will only change when the new generation comes in with new attitudes. When you get older, you get more entrenched in your views and you’re not willing to give up your ideas. You can’t convince the current generation of people in charge. But you can start convincing the younger generation. When they become professors, things will change. I think things will improve in the future.
SC: How do you show people that they will benefit from putting their work in the public domain and be practitioners of open science?
SV: What I’m doing is I’m trying to show this by example. All my data and code are online. People are using it to develop their own work. So I’m hoping that people will see that there is something gained by this. Another thing that I do is I publish re-analyses of published data, which is other people’s data. I do it in a constructive way to demonstrate some statistical point, and not to attack the original work. For example, I show that if you take this small sample study, and you re-do it, with a large sample, here is the gain in information. This is already having an impact; people are actually reading them and then they realize that they shouldn’t be running a small sample study, because they are losing information, and are being misled by their data. The new generation is actually reacting to this. I’m seeing more and more papers with power analyses being published, and people are actually running large sample studies. It’s amazing, just in the last few years.
SC: Yeah, because ultimately, it’s not about getting a significant or a non-significant result, it’s about having a result you can rely on.
SV: Right. And other people can build on your data. You can build up on information over time, even if you don’t have high powered studies. I also have a very narrow selfish gain here. If all the data were available, I could evaluate my model more accurately. So what happened to me was that we did a major data evaluation of my model and what we realized from that data analysis is that all the data was crap. There was so much uncertainty in the data that we couldn’t evaluate the model predictions. So then I started spending hundreds and thousands of Euros to try and redo these studies with high power. So that’s when I realized, why don’t other people do this? So I started to write methods papers, to demonstrate what happened if you ran a high-powered study, what you get.
SC: It’s a kind of a ‘better together’ approach and everybody benefits.
SV: Exactly. Also, science is incremental. I’m just a small drop in the ocean. I’m contributing a tiny bit, and other people are contributing their tiny bit. But together it’s going to build up to something.
SC: On the one side we talk about science and then there is also the public perception of science. Although much of research is paid for by the taxpayer, so little of it is actually understood by them. Do you think that scientists have an ethical obligation to explain what they do? Should the general public know about the statistical problems in academic research?
SV: I think definitely this should be communicated, but it has to be communicated in a sophisticated way. What journalists typically do is that they will sensationalize results or findings, like “Coffee causes cancer”, and it’s partly filtered through their own ignorance about the problem—making binary decisions from papers that they have half-understood, and also trying to make a story more saleable. What that does is distort the message quite a bit. The press releases that come out from the universities, they also contribute to this. If a journalist could actually provide a more sophisticated summary of what’s happening, definitely that should be communicated. Thankfully, many people are doing this right. For example, there’s this great book, ‘The Art of Statistics’, written by David Spiegelhalter, from the University of Cambridge. His books show us how you should teach people about risk. But it’s the journalists who have this responsibility. I can’t do that, because I’m totally focused in my own wild world studying my obscure problem.
But, all this has to be well understood by a journalist before they can write about it. So one of the problems for journalists I think is that the paper itself is usually written in a very misleading way. The authors are to blame for writing total garbage in their paper. Their conclusions are often wrong, you know, from their own analyses. And so then the journalist reads this famous researcher’s work, and now he or she has to draw their own conclusions about that. They have to have the skill set to do that and most people don’t have that. You need to be a statistician to understand that the work is garbage! That’s a barrier I think, to being able to communicate accurately. That’s why you get all these nonsensical things in the press.
What seems to be happening today is that any controversial or uncertain result can be hijacked in social media. And it’s very hard to control this today. I think that the only way that this can be controlled is if journalists take great care in presenting a nuanced story, and take great care in teaching people how to process uncertainty in the form of a conclusion. What does that mean? For example, with smoking it’s a clear case. Smoking causes cancer. But does coffee cause cancer? Or does coffee cure cancer? Or does coffee reduce the risk of cancer? It’s important for a journalist to actually explain that we can’t actually draw that causal link because drawing causal links requires certain standards to be met. And those standards are never met, because there are all these confounding variables. And there’s noise. We see some patterns and we don’t realise that those systematic patterns that we’re seeing could be just pure noise. Our brains are kind of designed to look for patterns, and it’s very hard to defeat that. You need training to defeat that thinking, and people don’t have that. Journalists can provide that.
SC: It’s not just that humans are innately bad at recognizing randomness. I think it’s also psychologically, emotionally satisfying to say we have a clear answer for this or a clear answer for that, and you’re taking that comfort away when you say “Actually we’re not certain about this at all but I can tell you how uncertain I am.”
SV: For me personally, it took me a long time to understand what uncertainty means. That was not something that I ever thought about in the first forty-five years of my life! It’s only recently that I started to think in those terms and that’s a radical shift in thinking. You’re not taught like that in school! I think trying to teach people to think in terms of uncertainty is a very hard thing. It needs to become more automated in their minds - not the point value but the uncertainty around the point value. So if I go to my doctor and my doctor tells me, look you have this horrible infection, and your risk of death is such and such, he’s telling me a point value. And I’m not interested in that point value. It doesn’t tell me anything. I often ask my doctor ... “how unsure are you of that?” They don’t even know what my question is!
SC: I think the least we can aim for is to impress on people that everything you do or say or conclude has a degree of uncertainty about it. That could be a massive revolution!
SV: That would be a very big insight, I would think. That takes time and it takes examples, and I am happy that we are trying to tackle that.