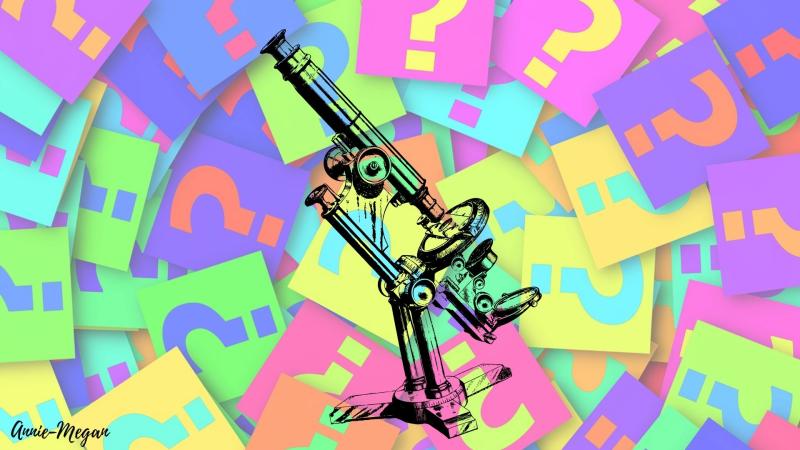
Jack: That, my dear Algy, is the whole truth pure and simple.
Algernon: The truth is rarely pure and never simple.
— Oscar Wilde in The Importance of Being Earnest
We live in a perplexing world, one where it is often hard to know what to believe. It has grown increasingly clear over the last few years that academic science is suffering from a ‘replication crisis’, where scientists have realized that a large number of published findings cannot be experimentally reproduced, and are almost certainly false. At the same time, even when scientific evidence on an issue like climate change is absolutely crystal-clear, some world leaders remain sceptical, and the national policies of those countries pay no heed to the coming catastrophe. Similar doubts, questions and threats haunt the world of clinical medicine.
If there’s one thing we need now more than ever, it is science-based political policy and a scientifically-literate lay public. In a world where more than half of all published studies are probably false, where companies sow doubt among their consumers about the safety of their products, and hysterical pseudoscience claims the lives of children, public scepticism about new scientific claims is not just desirable but necessary. It just isn’t enough to conclude that a thing must be so because “scientists did a study”. What was this study? How was it actually designed? Was that design statistically sound? Do the conclusions of the study actually follow from the data? Did the authors, or the people reporting on the study, have any conflicts of interest? Answers to these questions are paramount in separating the grain from the chaff.
Good science journalism often faithfully reports the facts in the studies, but possibly not as critical as they ought to be. Specifically, some aspects of the replication crisis, its origin, and the ongoing efforts to combat it, and more generally, questions of the scientific method, healthy scepticism and why scientists succumb to their all-too-human failings need to be viewed and understood objectively.
In a series of articles, Research Matters tries to explain the commonly accepted process of scientific methodology, the interpretation of scientific studies and the obvious pitfalls. It is hoped that this series will help lay public in analysing any understanding published scientific studies for what they are, instead of believing just because ‘scientists say so’.
Powering up: What makes a study ‘good’?
A fundamental point about a scientific method is to prove or disprove something. Unlike mathematics, where the path to a ‘proof’ is obvious, in most branches of science, the best we can do, as the late American paleontologist Stephen Jay Gould said, is to confirm something to such an extent that ‘it would be perverse to withhold provisional assent’. Every experimental study sets out with a hypothesis, an idea about something that might possibly be true, and then tries as rigorously as possible to disprove that hypothesis. The condition that might cause the difference is present in the test group, and absent in the control group. Statistical tests are then used to figure out how likely this actual difference is, given our assumption that the two samples are truly drawn from the same population.
The likelihood of this difference is captured by the much-revered and much-misunderstood p-value. As an example, let us imagine a medical researcher trying to see if a new pain medication helps relieve postoperative pain in patients. She has two groups of patients and gives one group the new treatment and the other the conventional treatment. She monitors the outcomes by a criterion she has decided on before—how often the patient wakes up at night due to pain, or a self-assessment of pain levels by the patients, and compares the outcomes for both groups of patients. She does her statistics, sees that the new drug does in fact reduce pain more effectively than the old one, and reports a p-value of 0.001.
What she is saying with this value is—“if there isn’t a real difference between my two groups of patients and the new drug doesn’t reduce pain, the probability that this outcome simply happened by chance is 0.001.” In other words, the p-value is the probability of obtaining a difference between a test group and a control group if we assume there is no difference. The smaller the p-value, the smaller the probability that there is no difference, and the more likely it is that we’ve observed a real difference. When the p-value produced by our statistics crosses an agreed-upon threshold, usually a value of 0.05, we conclude that our assumption, which was that there is no difference between the groups, was wrong, and congratulate ourselves on having found a true, ‘statistically significant’ result.
But not so fast! The p < 0.05 threshold might be taken for granted, but crossing it is simply not the acid test for the truth of a theory. By definition, it cannot be! Biological organisms, like ourselves, are terribly variable, and averages disguise distributions of values around them. For example, the average height of Indian women is 152 cm, but a woman might be 145 or 160 cm tall and still fall within the bell-curve that has an average of 152. What a p value of 0.05 really tells us is that, if there is truly no difference between the two groups, and we repeat our study many many times, 5% of those studies will falsely conclude that there is a difference. This is our alpha, or false-positive rate, where we conclude that there is a difference when really there isn’t.
The ‘p < 0.05’ is an arbitrary criterion, recommended with caution by Ronald Fisher, a British statistician who came up with the concept back in the 1920s. It has since been unquestioningly taken as the magic goal-post a study must reach so it can be published. Such a flawed principle inevitably resulted in a tremendous amount of bad science through practices like stopping a study as soon as the 0.05 threshold is crossed, the use of laughably meaningless statements such as “the results approach significance”, the practice of “p-hacking” where, for example, the experimenter randomly measures numerous different outcomes so that at least a few of them show up as ‘significant’ by sheer chance, the “file drawer” problem where non-significant results are simply forgotten and so on. Such practices are distressingly common in academic science, and are possibly being unquestioningly taught to new generations of graduate students every day. There has been a furious debate lately about the usefulness of the p-value, and if it should be scrapped completely. Whether the p-value lives, dies or survives in some other form, it is nevertheless important to understand what it means and why it matters.
If alpha is the false-positive rate, beta is the false-negative rate. It is the probability of falsely concluding that two groups have no difference, when they really do. When beta is subtracted from 1, we end up with the quantity known as ‘power’. When scientists talk about the power of a study, or say that a study is under-powered, this is what they are referring to—the probability of finding a difference between the two groups when that difference truly exists. When this probability is too low, then even a study that finds a large difference between its test and control group cannot really be trusted, because it has a high false-negative rate.
Alpha, beta, power and variance are all mathematically and conceptually related to another term that makes far more intuitive sense—the sample size of a study. It is the number of observations in a group. The general idea behind adequate sample size is familiar to us all—think of that one person you’ve heard of who smoked a pack of cigarettes a day and claimed until he died at the age of 94 that smoking couldn’t possibly cause cancer. Drawing general conclusions from tiny numbers is indefensible in both everyday life and in statistics for basically the same reason—a tiny number is more likely to be unrepresentative of reality.
With everything we now know about structuring a study, it should come as no surprise that a single study with a single positive finding, however well-designed, should never be regarded as the unquestionable truth. There are multiple factors that can introduce bias into a study and compromise it, and they can all play off and exacerbate each other. A crushing incentive in academia to publish papers or face massive career roadblocks; hiding papers behind expensive journal fees; a general misunderstanding of statistics; publication bias in favour of ‘positive’ results (“p is less than 0.05, so the experiment worked! Hurray, I can publish it now!”) and the disproportionate attention given to new studies over those that seek to recreate previous findings ...all of these have contributed to the present state of affairs.
“If you torture the data enough, nature will confess”
— Ronald Coase
It is crucial, however, that we distinguish between ‘unintentional’ factors like poor statistical analysis, negligence or lack of understanding, and deliberate fraud. In its way, fraud too is a wretched product of a system that values novelty and publication output over quality science, and its consequences can occasionally cross the line from wasted resources to outright tragedy. In 2014, Dr. Yoshiki Sakai published a study in Nature, which was later retracted when an investigation uncovered evidence of misconduct. Dr. Sakai killed himself shortly after, as did another Japanese scientist, Yoshihiro Sato, who was at the center of an even larger scandal involving hundreds of papers published with faked data. The issue of scientific fraud, where researchers intend to deceive with data-fabrication, plagiarism, falsification, deliberate misrepresentation and the like, is massive and deserves its own discussion.
The baby and the bathwater
Well then, what’s a scientist or a science-enthusiast to do? The recognition of the replication crisis implies that vast amounts of resources—time, money, careers, graduate students’ sanity—have all been spent in the pursuit of bad science. Experiments designed so poorly that their results can neither be trusted nor replicated are a waste. Far from advancing our understanding of the Universe, they are an active step backwards.
Academic institutions are as prey to human failings as any other, and as amenable to improvement through human effort. A large part of the solution lies in sound scientific methodology and journal policies free of conflicts of interest. The Open Science movement has risen as a result of the scientific community taking heed of this crisis, and it’s time the science-reading lay public became aware of it as well.
However, in spite of the prevalent replication crisis, we cannot write off the scientific method as ‘just another ideology’. Some might very well be tempted to do so, and just go back to ‘gut feeling’, ‘scripture’ or good old ‘spiritual intuition’ instead. But, the scientific method is possibly the best empirical method humans have to determine the truth about the world we live in. It has taken medicine, for example, from charms and amulets to antibiotics and Intensive Care Units. In fact, medical research provides some of the most striking examples of how things can go wrong when people distrust science—nearly-eradicated diseases come back thanks to vaccine scepticism, children are afflicted with lead-poisoning because their parents believe in alternative medicine, and thousands die because their governments do not believe in science.
Unconditional acceptance of the findings of every study is not scientific - indeed, the critical evaluation of data, far from being opposed to the scientific method, is an integral part of it. Both scientists and the lay public would do well to remember that.
This article is the first part of the series ‘The How and the Why: Interpreting Scientific Studies’, brought to you by Research Matters. The series focuses on the method of scientific studies, including emphasising the importance of meta-analyses, the repercussions of the replication crisis and the inclusion of ethics in experimental biology. We hope this series will better enable our readers to understand and evaluate scientific research they are interested in and those that could impact their lives.