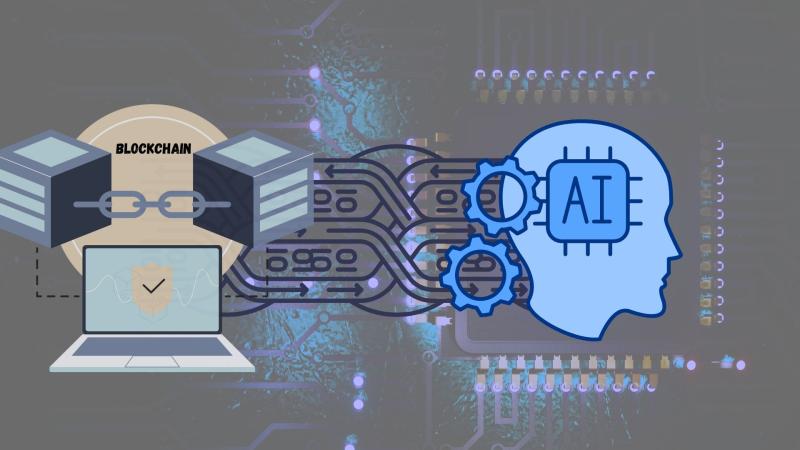
One of the primary concerns with the Artificial Intelligence (AI) boom is the privacy of the data used to train AI. With nations now keen on building more tailored AI and large language models (LLM), how we use and protect data is becoming paramount.
New research from the Indian Institute of Technology (IIT) Kharagpur has proposed using blockchain-based technology to share data securely to train an AI model. The researchers have improved Federated Learning (FL), a type of machine-learning model, by combining it with blockchain technology and other privacy measures. This combined approach aims to make machine learning models more secure and fairer for everyone participating.
At the core of their research is the Federated Learning model, which is trained across multiple devices without requiring raw data transfer. Like dividing a large project into smaller chunks to work on individual personal computers, FL works by allowing individual devices (like smartphones and PCs) to contribute to training an AI model without sharing the private data they hold.
In FL, individual devices or ‘nodes’ train a standard model on their local data and share only the results, not the actual data, with a central server. The data is usually encoded using Local Differential Privacy (LDP). This well-known privacy model adds a layer of 'noise' to the data before it’s shared, keeping the individual information private.
The researchers present a new approach called SBTLF (Secure Blockchain-Based Tokenized LDP Federated Learning) in the current study. They realised that by combining blockchain technology with federated learning and adding a system of tokens and Local Differential Privacy, they could train machine learning models more securely and effectively.
Blockchain technology, better known for its association with cryptocurrencies like Bitcoin, allows users to share data stored as tokens in a ledger in a decentralised and secure way. The researchers developed HyperLedger Fabric, a specialised blockchain framework used to manage data and transactions securely among different parties in a federated learning environment. It provides a distributed ledger that records all the local nodes' updates and helps ensure the entire process is transparent. It adds a security layer by preventing any single point of failure, which could occur if only one system controls the process.
Next, the researchers propose using a token-based incentivisation system to allow fair sharing and use of data. One major challenge with the FL model is getting participants to share their data willingly and ensuring they follow the protocols. In some systems, participants could send heavily obscured, and therefore less helpful, data and still access the benefits of the learning model as others who contributed more meaningful learning experiences.
The SBTLF method tackles the problem by introducing token-based incentivisation linked to the privacy parameter. Participants earn tokens by sharing less obscured (less noise-added) data. Clearer (less obscured) data earns more tokens. The tokens can later be used to access global model updates and reward participants who contribute more valuable data. Moreover, by encrypting the model updates and using blockchain for security, the risks associated with security attacks and a single point of failure are drastically reduced.
While this research opens many exciting possibilities for secure collaborative machine learning, setting up a blockchain-based federated learning system can be technically complex and require substantial computational resources. Introducing token-based incentivisation, which involves a form of trading, may also require policy-level interventions.
Nevertheless, the research provides a promising approach to making federated learning more secure and efficient. It introduces privacy tools and a clever reward system to ensure fair participation. The research can change how AI models learn from decentralised data safely and effectively by finding ways to ease implementation and improve resistance to different types of attacks. As the field grows, integrating blockchain and privacy measures could set high standards for global data security and collaborative learning.
This research news was partly generated using artificial intelligence and edited by an editor at Research Matters