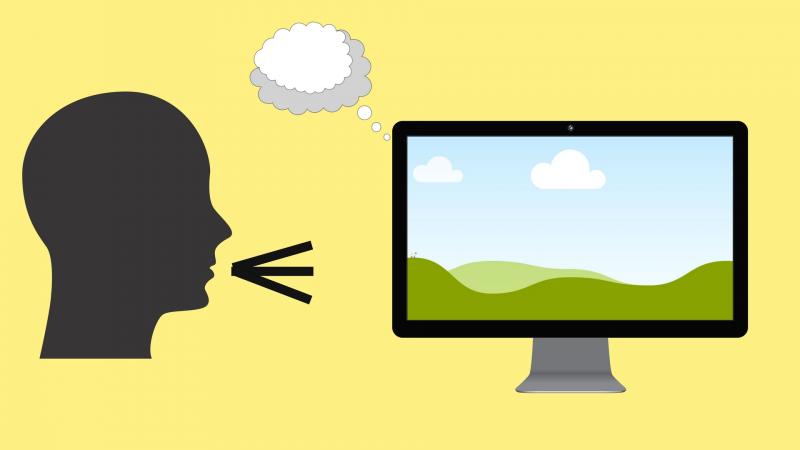
Researchers design a data-driven filtering approach that benefits machine understanding of speech.
Speech recognition software has dealt with its fair share of ridicule, what with the countless memes on its funny, and occasionally inappropriate, misinterpretations of certain voice commands. Intelligent personal assistants like Siri, Echo or Cortana find it all the more difficult to understand what we say in a noisy background, like in a car, at a restaurant, at the airport etc. Yet, we pursue our efforts to build the perfect speech-recognition program that one day will listen to everything we say anywhere. In this quest, where else can we look for motivation other than our brain -- the perfect ‘program’ that listens and understands a voice in different environments, such as train, street, etc. ?
And that is what Ms. Purvi Agrawal and Dr. Sriram Ganapathy at the Indian Institute of Science, Bengaluru have pursued. Their findings have been recently published in the Journal of the Acoustic Society of America, which indicates significant improvement motivated from neuroscience-based studies. They attempt to address the question whether big data can provide neuro-scientific cues for speech processing.
When a sound enters in our ear, different parts of the inner ear vibrate depending on the frequency of the input sound. This is depicted graphically in a Mel spectrogram, where the frequency of the sound is plotted against time and the intensity at each time-frequency point is color coded. "According to auditory neuroscience studies, it is hypothesized that such a spectrogram representation from the ear is transmitted to the primary auditory cortex in the brain which filters out the background noise and other irrelevant information and provides clean signals to the higher auditory pathway. Hence, humans are able to understand the speech content with ease, even in the presence of severe noise”, explains Ms. Agrawal. The human brain in the auditory cortex has millions of neurons with an architecture that enables the brain to process with highly flexible filters that can remove all possible types of noise including those which were not encountered previously. In addition, evidence suggests that humans are able to filter out noise even when they do not understand the underlying speech. Since this is incredibly hard to achieve in a machine, the question then is whether big data can help in designing such a filter.
In order to imitate this brain processing as closely as possible, Ms. Agrawal and Dr. Ganapathy use a convolutional restricted Boltzmann machine (CRBM) - an algorithm that learns the filters from the speech data on its own without any external label that teaches the algorithm what the correct output is.
A restricted Boltzmann machine (RBM) has two layers of nodes, which take in the input data (the first layer) and uses a set of binary nodes (the second layer) to learn the distribution of the data. The RBMs played a significant role in the early development of deep learning field where such models were used for pre-training large deep networks. With the enormous surge in data collection, these networks are not necessary in supervised deep learning. However, in this work, Ms. Agrawal and Dr. Ganapathy revisit the RBM model for filter learning.
A convolutional RBM (CRBM) is a variant of RBM that is more suited for learning filters from the Mel spectrogram. The CRBM learns filters that remove out the irrelevant part of the spectrogram. These filtered spectrograms act as the input features for a deep neural network (DNN), which has multiple layers of nodes to produce a more accurate prediction of the speech sounds for automatic speech recognition.
“The DNN is trained in a supervised fashion, meaning for each input speech file we give its corresponding text called as label. However, we observe that even with the lesser amount of labeled training data available for DNN training (semi-supervised training), the CRBM filtered features perform far better than the current speech recognition approach”, says Ms. Agrawal.
This algorithm was tested on a large pool of noise types – like restaurant, airport, car, street, train etc. In all these scenarios, the filter learning makes the speech recognition technology much more useful than what it is currently. Apart from this, the team tested the algorithm on reverberant speech - i.e., when the speech is recorded through a microphone that is far from the speaker - and found that it surpasses all other previous filtering approaches.We plan to extend our methodologies for Indian users, where there are several languages and accents. The current systems have a lot of trouble dealing with this heterogenous speaker population. However, humans are able to efficiently communicate in such a diverse multi-lingual environment. In the near future, we should be able to build machines that achieve the same as well.”, concludes Ms. Agrawal.