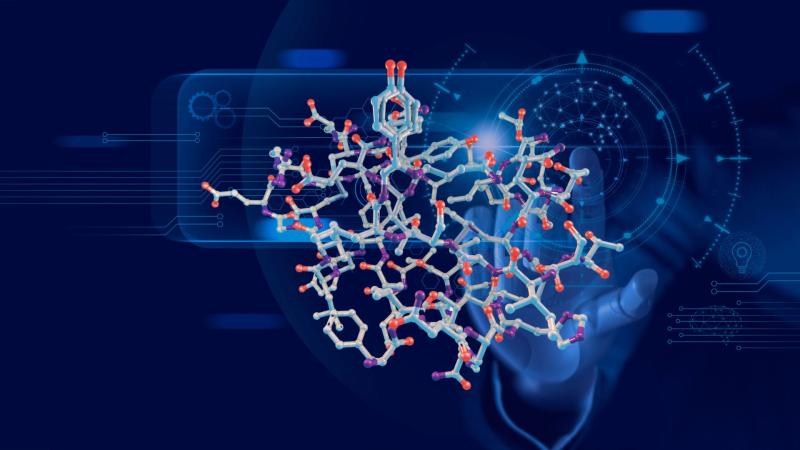
Proteins are essential building blocks of life. They play critical roles in nearly every process within our bodies, from supporting our immune system to ensuring our organs function correctly. But what happens when proteins change due to mutations and how do scientists predict if a mutation can be dangerous? Researchers from the Bhupat and Jyoti Mehta School of Biosciences, Indian Institute of Technology (IIT) Madras are diving deep into understanding how specific mutations in proteins can cause diseases like cancer. In a new study, they have catalogued and analysed all the available modern-day technologies and their potential use cases for diagnosing and treating protein mutations.
Proteins are complex molecules made up of smaller units called amino acids. Think of them like beads on a string, where each "bead" contributes to the overall shape and function of the protein. The sequence of these amino acids determines the protein's unique structure and role.
A mutation is a change in the sequence of amino acids. These changes can happen naturally over time or be triggered by environmental factors. While some mutations are harmless, others can alter a protein's function or structure, potentially leading to disease. For example, a small change in a protein associated with a disease like cancer can alter how it interacts with other molecules, leading to uncontrolled cell growth.
Researchers have been developing computational tools to predict which mutations might cause diseases. By studying large databases of protein sequences and their known mutations, they can identify patterns that suggest a mutation might be harmful. These tools use machine learning (ML), artificial intelligence (AI), and large language models (LLM), similar to how our smartphones predict the next word we want to type.
The researchers assessed several databases and tools to study disease-causing mutations in proteins. These resources provide information on genetic variants, some of which are associated with diseases, while others are considered neutral. Some of the key databases evaluated by the researchers:
COSMIC (Catalogue Of Somatic Mutations In Cancer): COSMIC is a comprehensive resource for information on somatic mutations in human cancer. It is invaluable for researchers looking to identify mutations associated with various cancer types.
The Cancer Genome Atlas (TCGA): TCGA is a large-scale project that collects and analyzes genomic data from different types of cancers. It provides detailed genomic information that researchers can use to study cancer-related mutations.
ClinVar: This database archives relationships between human genetic variations and clinical conditions. It provides a platform for researchers to access data about which mutations are considered pathogenic based on clinical research.
dbSNP: A database containing a broad collection of simple genetic polymorphisms, including single nucleotide polymorphisms (SNPs), which are important for understanding genetic diversity and disease association.
Apart from databases, the team also looked at applications and algorithms that can be used to predict disease-causing mutations, which can be used for real-world diagnosis, including AlphaMissense, an AI-based tool designed to classify human missense variants by analysing the potential impact of single amino acid changes (missense mutations) and predicts which variants are likely to be pathogenic; and Protein Language Models (PLM), models trained on large datasets of protein sequences to predict the effects of mutations using machine learning techniques to understand the functional consequences of changes in protein sequences.
These tools and databases serve as foundational resources for developing computational methods capable of predicting which mutations in proteins might lead to diseases. They provide the necessary data and analytical frameworks to understand the complex relationship between genetic variations and their impact on protein function and disease pathology. Moreover, these tools go beyond just listing potential harmful mutations. They assess how these changes might affect a protein's shape, stability, or the way it binds to other molecules. For instance, if a crucial interaction point on a protein is altered, it could impact processes like how a virus enters a cell, as seen with SARS-CoV-2, the virus causing COVID-19.
For their study, the researchers not only examined what these applications were capable of but also studied their limitations and offered possible solutions to improve them. The study offers a comprehensive look at what technology offers in terms of diagnosing one of humanity’s oldest and toughest challenges—cancer.
Despite these advancements, predicting disease-causing mutations is not without its challenges. One major limitation is the incompleteness of existing data. Many databases rely on samples from specific populations, which might underrepresent certain groups or conditions. Machine learning and AI models trained on this data can lead to biased predictions. To address these challenges, researchers are continually working to refine their models and expand their datasets with broader data collection and integrating more data types
The research into how mutations in proteins lead to diseases is a rapidly evolving field. With the help of technology, scientists are making strides in predicting these changes and leveraging this information to improve medical care. While challenges remain, the potential benefits of these discoveries are immense, offering hope for more effective treatments and better health outcomes in the future.
This research news was partly generated using artificial intelligence and edited by an editor at Research Matters