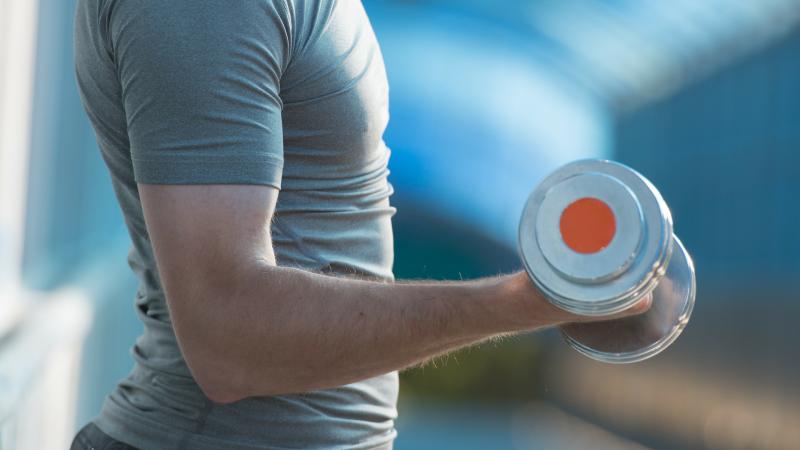
Researchers from the Indian Institute of Technology, Madras, have proposed methodologies that can improve the design of automated systems to detect muscle fatigue. In a recent study, published in the journal Computer Methods and Programs in Biomedicine, the researchers have used surface electromyography (sEMG), a technique which can record electrical signals over the surface of the skin.
Anyone too enthusiastic about lifting weights on their first day in the gym is aware of ‘muscle fatigue’. It is a condition in which one’s muscles are unable to generate enough force. It is observed not only in people who undergo vigorous exercise, like sportspersons, but is also a common syndrome in neurological disorders like multiple sclerosis, Parkinson’s disease and stroke.
In this study, the researchers instructed volunteers to perform bicep curls by lifting a 6-kilogram dumbbell until a point when they experienced fatigue. Bicep curls are dynamic contractions where the joint angles and degrees of muscle contraction keep changing, unlike isometric contractions (like the plank exercise) where the angles and degrees do not change. Most previous research has focused on isometric contractions, which involves less noise and variance in the sEMG signals. In contrast, dynamic contractions provide a more significant challenge to analyse. The researchers continuously recorded the sEMG signals from the volunteers’ arms during the exercise.
The time-varying signals collected during the first bicep curl (normal muscle condition) and the last one (muscle fatigue condition) have different frequencies inside them, just like a musical chord has different frequencies of its constituent notes hidden inside it. The researchers used a signal processing technique called Time-Frequency Domain (TFD) transformations to extract these changing component frequencies. However, these powerful techniques spit out too many of these component frequencies, all of which might not be equally useful to classify the signal as being normal or not. So, to find out which are the best components, they used a method inspired by biology called the ‘genetic algorithm’ that starts through a set of features, allows the more ‘fit’ solutions to methodically change (‘mutate’) and give birth to fitter offsprings - and this continues till satisfaction.
The researchers then used other techniques that can guess, from these selected features, whether we have a normal or fatigued condition. The most accurate among the methods tried out was the Support Vector Machine (SVM), which imagines the transformed signals from each bicep curl to be like a point in some space and draws an imaginary boundary which can best classify the points while keeping maximum distance from either type of point. Using SVM with four selected features gave a high (91%) accuracy in detecting muscle fatigue. Besides, the researchers claim that these accurate and fast techniques can also be used in the much simpler-to-analyse isometric conditions too.
With the techniques mentioned above, one can imagine easier detection of fatigue at a personal level, which can not only serve as a measure of improved human performance, but can also help in preventing slip-induced falls and injuries in occupational settings, sports settings, or rehabilitation programs.