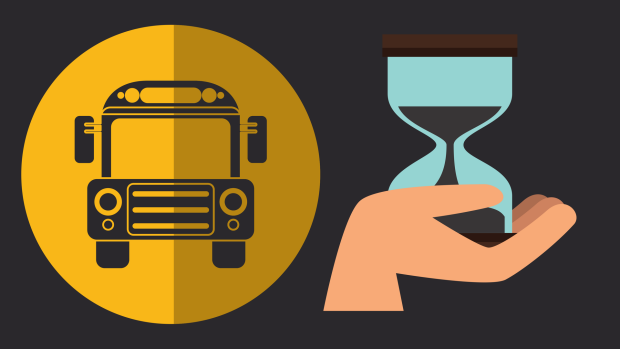
Illustration: Siddharth Kankaria / Research Matters
Haven’t we, at times, been late for an appointment because of the unreliable public transport? Waiting for a bus that may never turn up is not an unusual experience for those who use public transport daily. The increase in vehicular population adds to the woes, creating a traffic snarl that has become unmanageable anymore. While building new roads and flyovers may divert a part of the problem, a sustainable solution to the traffic pile-up in cities lies in encouraging the use of public transport. How can technology help the public use an ‘unpredictable’ transit system with convenience?
In a recent study, Anil Kumar, Dr. Lelitha Vanajakshi and Dr. Shankar Ram at the Indian Institute of Technology, Madras (IIT-M) have tried to address this problem. Using techniques of data science, they have devised an application with a simple user interface that accurately predicts the arrival and the travel times of Metropolitan Transport Corporation (MTC) buses in Chennai, Tamil Nadu.The web application developed by the team shows the estimated arrival time of a bus at a particular bus stop, and includes a feature to track the bus on a map.
The main objective of this study was to analyze the time of travel and determine what kind of inputs would best predict the travel and arrival times of the buses. From this data, a bus travel time prediction system was developed based on the concepts of ‘time discretization’. “In time discretization, the evolution of travel time within a given subsection will be studied over time to predict the next travel time”, explain the researchers. In other words, instead of using the time taken to travel between two points on a route to predict the travel time for subsequent points on the route (also called space-discretization), the researchers used the travel times between the same points from past days, weeks, and months to predict the current travel time.
The researchers studied two major bus routes of MTC - 5C (spanning 15 km, connecting Parry’s to Taramani) and 19B (a 30-km route, from Saidapet to Kelambakkam) – plying in the most congested parts of the city. They used Global Positioning System (GPS) to track the two routes and collected the travel times for 2227 trips over 45 days. They further divided the data into four groups based on the time of the day - morning off-peak, morning peak, afternoon off-peak and evening peak. They then tried to derive a pattern based on the data collected that could help in accurate prediction.
The collected data was also split into input days (30 days) and output days (15 days) in order to carry out a ‘t-test’.“A t-test is a very commonly used statistical hypothesis test used to determine if two sets of data are significantly different from each other. In this study, the t-test has been conducted for the difference between the mean of two independent samples (input day and output day). Here, the input day refers to the trips that happened during the input period and output day refers to the day being analyzed”, explain the researchers.
Once the travel time pattern was obtained, the researchers used another technique called ‘Kalman filtering’ to predict the arrival and travel times of buses in the two routes. Kalman filtering is an algorithm that uses past travel times to produce estimates of certain variables that are vital in calculating the travel time of the current trip.
When compared with space-discretization, the researchers found the time discretization to be more accurate in predicting current travel times. In particular, while estimating the time taken to cover a part of the route, the former was off the mark by 75 seconds whereas the latter deviated only by 32 seconds. Even in worst-case scenarios, the time-discretization method was found to be superior and accurate.
The researchers believe that there are still a few ways to make the prediction system more accurate. “The accuracy of the proposed method can be improved by also including the mean and the variance (the deviation between samples) of the measurements and process noise of travel time in each subsection separately. The process noise is related to the changes in the travel time, which is random”, add the researchers on the future direction of the research.
With the team’s new user interface telling us when the next bus is going to pull into the stop, it might become much easier to depend on public transportation. No more cursing the unpredictable traffic, thanks to this team from IIT Madras!