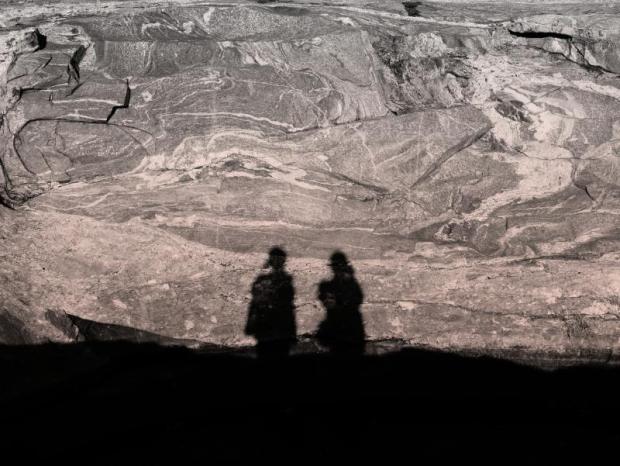
Photo: Siddharth Kankaria/Research Matters
Recruitment process is a time-consuming affair and a lot of research is underway in automating some of the aspects of this process. Computer-based tests that evaluate an individual’s knowledge are rampant. But can such tests be developed to measure the communication skills of a candidate, in place of a face-to-face interview? Prof. Dinesh Jayagopi and his team of researchers at the International Institute of Information Technology (IIIT), Bengaluru, have now identified features and attributes that help in predicting the communication skills of candidates. Using Machine Learning, they have developed a prediction algorithm that can evaluate the communication skills of an individual. “The goal of the study is to put a candidate in both manual and automated test settings and compare their behavior and behavior perception across the two scenarios”, explains Prof. Jayagopi.
The researchers first collected videos of 106 candidates for the two settings – face-to-face interviews and computer-based interviews. Three interviewers rated each candidate based on attributes such as fluency, rate of speech, enthusiasm, effective communication, etc. in both settings. The ‘Kappa coefficient’, a statistic that measures inter-rater agreement for categorical items, was used to measure the agreement among the three interviewers on each attribute. A higher value of the coefficient indicates higher agreement for ranking an attribute among the interviewers. The fluency attribute kappa coefficient was found to be 0.79, while physical appearance received 0.1, which proves that that particular attribute is subjective.
From the attribute ratings of the attributes and the values of the Kappa coefficient for each attribute, the researchers also realized that the participants behaved differently in the two environments. In a face-to-face interview setting, they were more communicative, while in a computer-based interview they were more expressive due to the fact that there was no one monitoring their behavior. This answered one of the questions of the study - are candidates perceived to have different behavioral tendencies depending on the environment.
Audio features such as the tone, pitch and style of the candidate’s voice, called as prosodic features, and visual cues like gestures, nodding of the head, and the duration of candidate’s smile, were extracted from the video clips using automatic feature extraction software tools. A combination of features was extracted to represent an attribute. For instance, lexical features like the length of an average sentence and the number of unique and difficult words in a sentence were used to indicate the fluency of the candidate. Also, interviewers manually rated attributes while features were automatically extracted from the videos. “When features are very good, we should expect to reach feature based prediction accuracies as high as attributes”, explains Prof. Jayagopi.
Based on the identified features and attributes, the researchers built a prediction system that would rate the communication skill of the candidates using Machine Learning, a concept where given a set of input and output data, the computer learns the relation between the two. The algorithm is first given a ‘training data’, so it can ‘learn’ the relation. Then it is given the ‘test data’ to check how accurately it can predict the output. In this study, the system classifies the candidate as either “below average” or “above average” using Support Vector Machines, a Machine Learning classification algorithm, with accuracies of 88% and 92% in the computer-based and face-to-face interviews respectively using just the attributes as inputs.
Then, they fed features to the predictor and analyzed which particular features achieved high rates of accuracy. For computer-based interviews, it was found that prosodic features were able to give us 79% accuracy. In the case of face-to-face interviews, lexical features achieved 83% accuracy but prosodic features still achieved a whopping 80% accuracy. Thus, the predictor indicated that people who spoke with great energy and had a good rate of speech, both of which are prosodic features, were generally good communicators.
The researchers believe that a computer-based interview alone would be enough to categorize the below average candidates since the prediction accuracy in both the scenarios were almost the same. Once implemented, this system would automate the entire shortlisting process. “With our study we can safely conclude that our model will generalize to the Indian engineering student population. It remains to be seen if it will work in Indian non-engineering-student settings and non-Indian populations”, concludes Prof. Jayagopi.