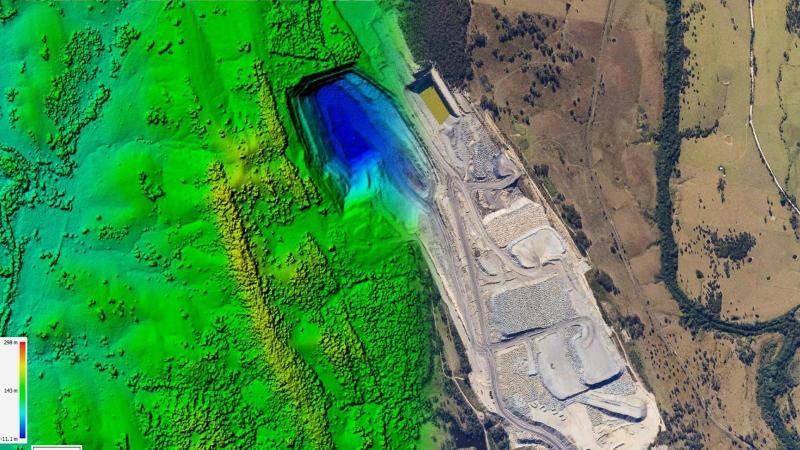
Image Source: Wikimedia Commons
Land surveys are used to find and monitor land features in large areas. Information such as differences in elevation, area and heights of buildings, vegetation, water and roads can be gathered by physically surveying the land. The same information can be gathered using pictures captured remotely by cameras mounted on satellites or tripods, drones and helicopters.
Another way to get the information about land features is to use sensors to capture data and reconstruct a three-dimensional representation via specific techniques such as lidar (Light Detection and Ranging). Aerial surveys cover large areas, reduce fieldwork, are less time consuming and take repetitive measurements to study how land usage changes over time. The data collected through aerial surveys is vast due to many measurements associated with any point on the ground.
Prof Surya Durbha and his team from the Centre of Studies in Resource Engineering, Indian Institute of Technology Bombay (IIT Bombay) have proposed and evaluated a novel computational method, named LidarCSNet, to compress lidar data and reconstruct a three-dimensional representation using the compressed data. Their method saves memory, time and computational cost, with negligible error compared to the reconstruction when raw lidar data is used without compression. The study was published in the ISPRS Journal of Photogrammetry and Remote Sensing.
Lidar is a technique that uses light pulses to gather information about land features which can be used to create a three-dimensional representation. A device installed on helicopters, drones, or tripods sends light pulses to the land and receives reflected signals from the ground under scrutiny. Depending on the time delay between the received and sent pulse signals, the number of reflections, and the intensity of reflected light pulses, a 3D representation of the region can be created.
The lidar data is huge. It needs large storage memory and heavy computations to extract information about the area surveyed. Using lesser data points can reduce time and resource costs, but it compromises the accuracy of the 3D representation. The challenge lies in creating a representation that is very close to the original, using minimum data points.
LidarCSNet uses a novel combination of computational methods called Compressive Sensing and Deep Learning to compress and reconstruct 3D lidar data. Compressive Sensing(CS) reconstructs information using fewer measurements or data points. Deep learning methods are machine learning algorithms that help ‘train’ the software to identify specific features in large datasets like audio, images, video. The researchers implemented LidarCSNet as a stack of software modules where the layers work one after the other to yield a more accurate result. This design is easy and scalable to implement for large amounts of data and avoids iterations, unlike earlier implementations.
The team demonstrated the efficiency of LidarCSNet on two pre-captured 3D lidar datasets for forest and urban environments in the Philippines. These publicly available datasets from the Training Centre for Applied Geodesy and Photogrammetry (UP TCAGP) and the PHIL-Lidar Program of the Philippines include the on-ground survey data for the region.
Reconstruction using LidarCSNet
The researchers used LidarCSNet to generate four compressed representations of the lidar datasets using different compression levels by choosing 75%, 50%, 10% and 4% samples from the total lidar samples. They used LidarCSNet to reconstruct the 3D scene from the four compressed representations for forest and urban data sets and validated the results against the on-ground survey data. In both case studies, they found that as the number of samples reduced, the reconstruction deviated more from the original, and the reconstruction time decreased.
Though the reconstruction was faster at 10% and 4% samples, its deviation from the original model could be unacceptable for applications that need higher accuracy, like autonomous vehicles. The researchers believe that their findings related to the reconstruction accuracy and reconstruction time for the different compression levels can help applications decide how much compression they should use to achieve the desired speed and accuracy. The findings can best help optimise remote sensing applications that analyse vegetation, forests and urban areas.
“Though LidarCSNet can be used with any lidar data, it will be more beneficial to use it in lidar applications like remote sensing where data is huge, rather than applications like autonomous vehicles where computation happens on a smaller data,” says Mr Rajat Shinde, an author of this research.
Land Feature Identification
The researchers demonstrated the goodness of LidarCSNet-reconstruction by using the reconstructed data to classify the land features in six classes of land-cover (ground, low or medium or high vegetation, buildings, bridge deck) and finding the accuracy of classification. They developed two novel classification frameworks called LidarNet and its enhanced version, LidarNet++, tuned to handle features of the various land classes. LidarNet++ gives a more accurate classification at the cost of more computations and time.
The researchers used LidarNet and LidarNet++ to classify uncompressed lidar data and the lidar data compressed and reconstructed using LidarCSNet at different compression levels. They compared the classification results with those obtained using other existing lidar classification methods. LidarNet++ gives the highest accuracy of classification among all the methods, on an average over all the six land classes and at all compression levels. For example, LidarNet++ classifies 86.43% of the points accurately when 75% of the samples were used. However, other frameworks could not achieve this accuracy even when all of the samples were used.
Prof Durbha says, “Having compression of data using LidarCSNet framework at the time of capture, on-board the capturing hardware in drones, would be very useful. It will eliminate the need to store or work on the huge raw lidar data and make it possible to use only fewer data points at the required compression level.”
The researchers feel that this work can immensely help lidar applications to optimise their speed and cost when surveying for the changes in land use and also in computer vision when building models of the surroundings. “We are working to make LidarCSNet available in a ready-to-use software format for other applications to leverage, after which it can be made open for others to use,” says Mr Shinde.
This article has been run past the researchers, whose work is covered, to ensure accuracy.