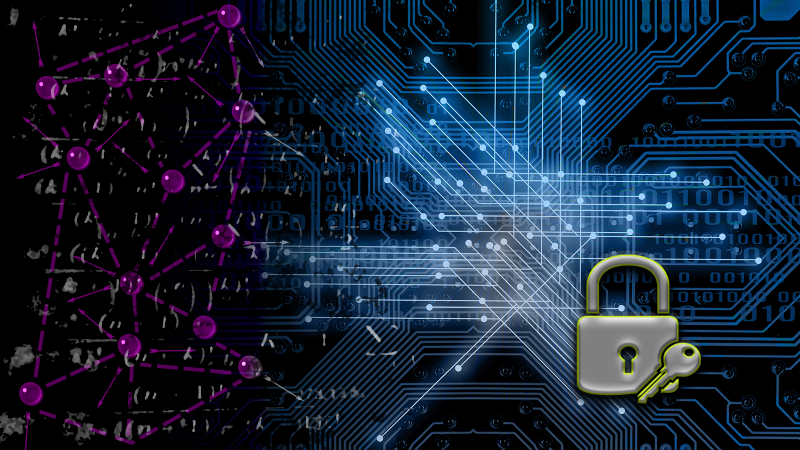
Solid particles suspended in a fluid are bombarded on all sides by particles of the fluid. They change their direction of motion haphazardly, and the distances they travel between one such collision and another are not predictable. Hence, their motion is said to be random. Discovered by the botanist Robert Brown in 1827 and named after him, Albert Einstein theoretically explained ‘Brownian motion’ in 1905. According to the theory, it is impossible to trace the motion of individual particles of the solid, which introduces inherent randomness to the particles’ motion.
Generating a truly random sequence of numbers holds the key to secure encryption of data. However, the task remains a challenge. Although computer scientists rely on several numerical techniques to generate random numbers, the methods always introduce a small degree of predictability in the sequence. In a new study, researchers from the Indian Institute of Science Education and Research, Kolkata, have used the naturally occurring Brownian motion to generate random numbers. Funded by the Department of Science and Technology and Ministry of Human Resource and Development, Government of India, the study was published in the journal Frontiers in Physics.
The researchers first suspended plastic particles about 3 microns in diameter in a mixture of water and common salt. They tracked the individual particles using a laser focused on these particles, at a rate more than thousand per second. The laser they used does not affect the Brownian motion of the particle it probes, relying on the invention called ‘optical tweezers’, which was awarded the Nobel Prize in 2018. The researchers recorded the position of the individual particles in a computer and studied their motion.
Any instrument used in the laboratory first needs calibration –– that is, the parameters that determine the instrument’s behaviour need to be quantified to enable reliable measurements. First, the researchers used the position of the individual plastic particles to calibrate the optical tweezers. They used a numerical technique called ‘machine learning’ on the data they had collected. It first used a section of the data to identify the numerical parameters responsible for driving different kinds of motion of the particles. Then, it used the rest of the data to identify those parameters that accurately described the motion they had observed. The researchers demonstrated that indeed, the optical tweezers they used were behaving as expected. When small anomalies arose, the researchers showed that these deviations would not affect the data they recorded for the particles’ motion.
The researchers used their machine learning technique to demonstrate that the plastic particles indeed undergo random motion in the salt-water solution. They showed that when they measured the parameters relevant to the motion using their method, the results match those of traditional techniques, such as the Fourier transform, named after its discoverer Joseph Fourier. Their approach, however, requires minimal human interaction.
“Thus, we provide new insights on calibrating Optical Tweezers,” says Raunak Dey, the lead author of the study.
The researchers then used the trajectory of the individual particles to generate a sequence of numbers. They conducted a series of 15 standard tests of the randomness of a sequence of numbers. “The tests tell us whether a string of numbers qualifies as random, and also allows us to judge the extent of randomness,” shares Raunak. The researchers concluded that indeed, the numbers are random up to a high degree of confidence. They further showed that if the rate at which the laser observes the particles is higher, it gives them increasingly more certainty about the randomness of the sequence. “We have directly borrowed these random numbers from nature instead of relying on artificial algorithms,” adds Raunak.
The numerical technique also enabled them to independently calculate the parameters of the fluid in which the particles moved, like how much internal friction it offers for its flow. They demonstrated that these independent measurements agree with the well-known values for the fluid. It can have interesting implications. Measuring fluid parameters is difficult for certain biological fluids, like living cells, or fluids which sometimes behave like a solid, like molten lava.
“It is difficult to use conventional techniques to study these special fluids, but we can now apply our technique on these systems,” Raunak signs off.
This article has been run past the researchers, whose work is covered, to ensure accuracy.