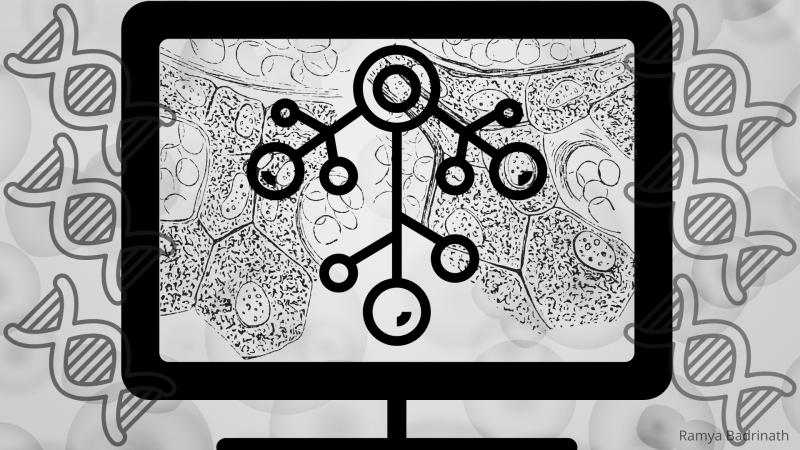
In a recent study published in Nature Communications, researchers have described a new statistical method, ‘LinTIMaT’, for reconstructing cellular lineages, giving scientists the ability to deduce the evolution of cells in a biologically growing system.
Cells are like us; they are fully functioning units that have evolved from their ancestors. And so, just like us, they too have a family tree, a lineage. Scientists explore the relationship between cells and their ancestors using ‘phylogenetic trees’ to find answers to questions in the biology of organism development, various diseases, and more.
Construction of phylogenetic trees requires knowledge of cellular lineages – which cell evolved from which and how. Describing cell lineages is challenging because even the simplest of organisms have thousands of cells. Describing how every single cell evolved from its ancestral cell would require a whole lot of data. And this is where high-throughput sequencing technology and statistical analysis comes into the picture.
LinTIMaT integrates multitudes of mutation and gene expression data to reconstruct cell lineages using statistics and machine learning. The algorithm delivers its results with better accuracy than the existing methods, which rely on mutation data or gene expression data independently.
LinTIMaT’s likelihood-based method will undoubtedly be promptly picked up by computational biologists across the globe for its practical applications, which are aplenty. Take, for instance, its applications in cancer. Cancer tissues are made of diverse cell types all likely having originated from a single rogue cell. Cell lineage maps of cancerous tissues will allow physicians to make informed calls on drug courses that will work best on a particular line of cells. The applications are umpteen.
It was in September 2018 that Dr. Hamim Zafar, now an Assistant Professor at IIT Kanpur, visited Carnegie Mellon University to work as a postdoctoral Fellow. Dr. Zafar came with expertise in Computer Sciences and building phylogenetic trees using DNA sequencing data from single cells. Sequencing is unravelling the order of nucleotides in DNA or RNA using a handful of chemicals and computer algorithms.
During his research at Carnegie Mellon, Dr. Zafar realised that single-cell RNA (scRNA) sequencing technologies were now gaining popularity for its application values across life sciences and medicine. For this reason, he wanted to apply his competency on single-cell RNA sequencing data, and that is when he stumbled upon the problem – there were some drawbacks in the existing methods of reconstructing cellular lineages. He and his postdoctoral mentor Prof. Ziv Bar-Joseph, figured out that combining mutation and expression datasets could be the answer. Dr. Zafar teamed up with Chieh Lin, a student in Prof. Bar-Joseph’s lab, and together they developed LinTIMaT and began exploring its potential. Following his postdoctoral stint at Carnegie Mellon, Dr. Zafar carried the project across the seas to IIT Kanpur, where it saw its completion.
The dataset that LinTIMaT uses is of two kinds. The first is a mutation data set, which works with the data derived from cells that have marker arrays introduced into them. As the cells divide and evolve, these arrays accumulate mutations, in this case introduced by a CRISPR-Cas9 system, a widely popular genetic engineering system. The mutation data, including ‘which cells have acquired what mutation at which stage of their development can tell us a lot. Scientists collect information from thousands of cells and feed them into a computer algorithm to make sense of the data.
The second kind of data that LinTIMaT uses is gene expression values. This is scRNA sequencing data that provides RNA expression profiles of individual cells. The data, which is again high-throughput, provides a glimpse into understanding cell type and thus allows better fitting of unmatched pieces of the cell lineage jigsaw better into the puzzle. This data helps resolve the obscurities observed when lineages are interpreted based on mutations alone.
Experimental biologists tend to use Maximum Parsimony, which is a classical, off-the-shelf, method for phylogenetic tree building, and although it is highly valuable, it does have its shortcomings.
“It reconstructs lineages based only on genetic markers. With such an approach, we will not be able to recover some branches (of the cell lineage), because they are not supported by any genetic markers with mutations in them and so what we get is incomplete information. Another drawback is that we cannot integrate data across individuals using genetic markers, which are completely random”, explains Dr. Zafar.
scRNA sequencing data is currently being used for inferring the differentiation trajectory of cells. Still the problem is that what you get is not the exact genetic lineage but a representation that shows how gene expression values change from one cellular state to another. Although the methods do have their merits, one cannot ignore the limitations.
“With LinTIMaT we can circumvent these drawbacks and reconstruct cell lineages more accurately than the methods currently being employed, in a single computational framework”, Dr. Zafar asserts.
By integrating single-cell transcriptomic data, LinTIMaT patches the loopholes in the cell lineage landscape derived from the mutations data alone.
“It was the most exciting to see that actually using these two different sets of information, which no one appears to have attempted before, was indeed giving us gain over the existing methods”, he expressed.
Also, LinTIMaT allows the integration of numerous individual lineages for the reconstruction of a coherent lineage tree.
“I am now looking forward to diving deep into the model in a more comprehensive manner that will improve the result beyond what we are getting now”, he signs off suggesting his intentions to proceed with optimizing the platform further.
This article has been run past the researchers, whose work is covered, to ensure accuracy.