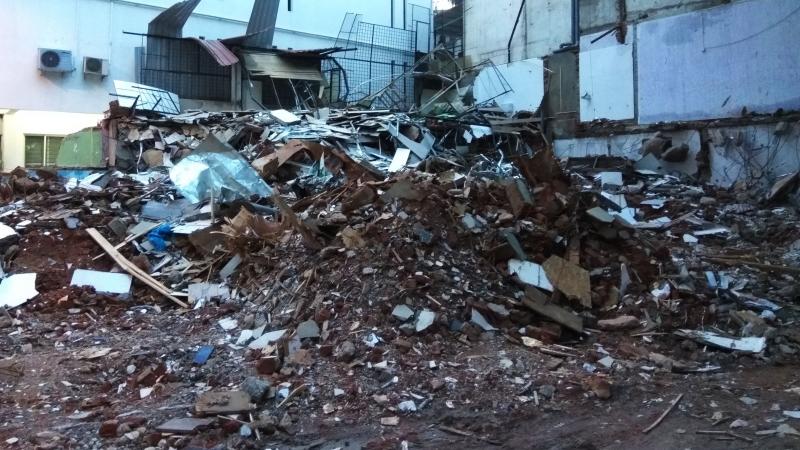
When a disaster strikes, every moment that is saved could help save a few precious lives. Now, a recent study by researchers from the Indian Institute of Technology Kharagpur has proposed a mechanism in which faster, cheaper and personalised response could be provided to the victims during disasters with the use of Wireless Body Area Networks (WBANs).
A wireless body area network is a network of sensors connected to the human body, which generate a variety of data related to one’s health, including pulse rate, blood pressure, temperature and other vitals at periodic intervals. The collected data is then transmitted to a centralised processing system that can analyse and can direct health-care professionals to take appropriate actions.
In a post-disaster scenario, where immobilised victims are typically concentrated in crowded relief camps, multiple medical signals transmitted by WBANs could congest the network. As a result, the healthcare professionals attending these victims remotely would lose out on getting accurate readings from many patients.
The researchers of the study suggest creating disease-specific groups of patients based on the medical data to address the above challenge. They have used models from social network analysis which can group and divide people based on the kinds of diseases prevalent and the likelihood of them spreading. The suggested mechanism works well in containing the spread of communicable diseases like cholera which are common in the post-disaster period due to the non-availability of clean water.
The proposed improvisation helps healthcare workers cater to the most critical groups first, and based on their expertise these workers can be connected to the neediest patients. For example, a cardiac specialist can address the group having the highest incidence of heart-related issues, and an orthopaedic could treat the group with patients suffering from blood loss or trauma.
Through simulations, the researchers have also shown that the proposed model is cost-effective with minimum delay, and has higher network reliability than existing models.