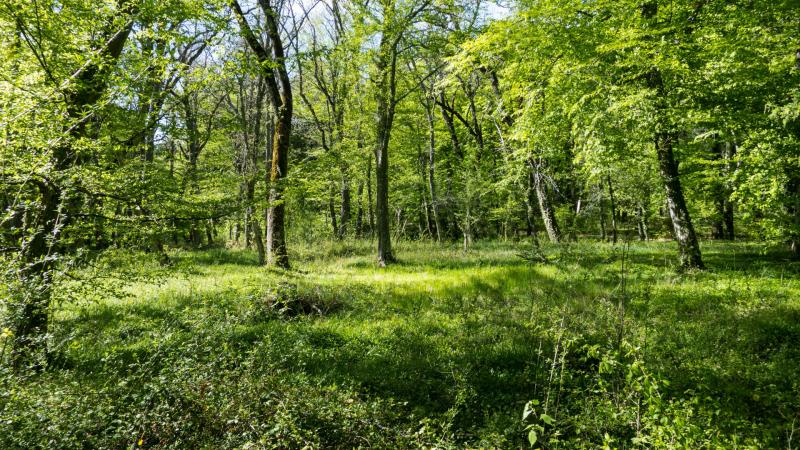
Researchers from Indian Institute of Technology Bombay, Mumbai and Cornell University have been reviewing the models and simulations used to study interactions between humans and natural systems. Their study reveals the reason why early prediction of an approaching tipping point of an ecosystem still remains challenging.
Human inadvertently affect the environment around them through their activities. Either by releasing pollutants or by diverting natural resources, ecosystems may be completely destroyed or protected depending on the management policies that are followed. Ecosystems like lakes, rivers and forests are all at the mercy of its human neighbours, with our actions having direct consequences to the ecology in these systems. Often a transition of an ecosystem to an undesired state, like eutrophication of a lake, can be fast, allowing almost no time for precautionary measures. Such instances can of course be avoided if we could predict such a tipping point well in advance, and take the necessary precautions. However, as an ecosystem is made of numerous entities interacting with each other, an accurate prediction of such a tipping point is a challenge.
“Often, there are considerable uncertainties that make identifying the threshold challenging. Thus, rapid learning is critical for guiding management actions to avoid abrupt transitions” remark the authors of the study.
Researchers often use models to study such complex systems, by identifying as many variables as possible to approximately predict its behaviour. In the new study, researchers looked at four commonly used data assimilation schemes and their ability to predict the eutrophication (over-enrichment of water by nutrients) of a lake. “In order to demonstrate the complex interactions between management strategies and the ability of the data assimilation schemes to predict eutrophication, we also analyze our results across two different management strategies governing phosphorus emissions into the shallow lake” explain the authors.
The four schemes studied were: ensemble Kalman filtering (EnKF), particle filtering (PF), pre-calibration (PC), and Markov Chain Monte Carlo (MCMC) estimation. Although the four models differ in their workings, they are based on a common framework—Bayes theorem, which describes the probability of an event occurring based on prior knowledge of the conditions that might affect the event.
The study reveals that when large amounts of computation is involved, EnKF, PF, and MCMC are similar in accuracy when it comes to predicting the amount of phosphorus absorbed into the lake. While EnKF and PF provided good estimation even at low computational cost, MCMC method provided the most accurate estimation of events, but only after strong evidence for an impending transition emerged from the observation.
However, all the four data assimilation techniques could only predict a tipping point, like eutrophication, only when such an abrupt transition was becoming inevitable. This makes predicting an abrupt change a challenge, until it’s too late. “Overall, we find that learning rates are greatest near regions of abrupt transitions, posing a challenge to early learning and preemptive management of systems with such abrupt transitions” conclude the authors, pointing towards a need for better schemes to model the human-nature interactions.