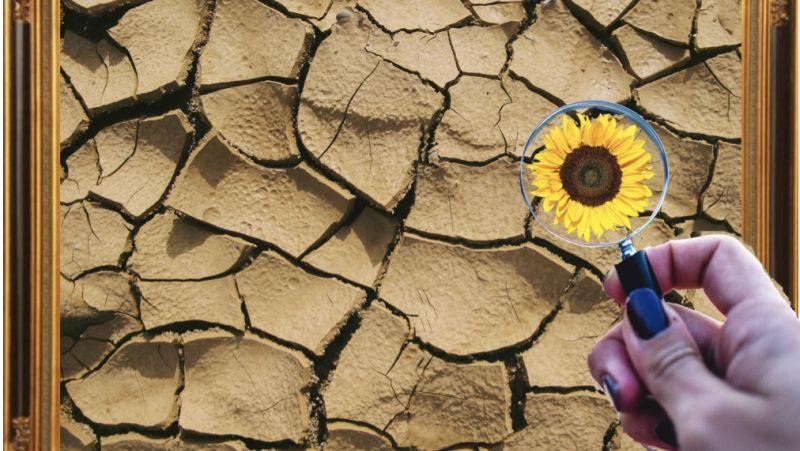
A statistical bird’s-eye view
One of the first things a graduate student new to a field does is acquaint oneself with the relevant literature. More often than not, a supervisor will point the student in the direction of a review, preferably recently published, and tell them to start there. ‘Review’ is a catch-all term however; in 2009 a study by Grant and colleagues used a simple analytical framework to identify fourteen different types of review and another related study from 2017 identified nine. All these types of reviews are not necessarily mutually exclusive. Systematic reviews, for example, comprehensively search for all the evidence pertaining to a subject and synthesize it into a narrative; critical reviews do the same but also critically examine the evidence and might even suggest models or hypotheses for future research.
Fundamentally, a meta-analysis is a type of review that gathers together all the data on a particular question, puts those data together into one large study and analyzes them to arrive at a conclusion that’s likely better-supported than smaller, isolated studies. Gene Glass was the first to come up with the term ‘meta-analysis’ and recognised the need for it, as even in 1976, he could write: “The literature on dozens of topics in education is growing at an astounding rate”.
A scientist doing a meta-analysis first figures out precisely what question she’s interested in, and what particular statistical results she will examine. She selects the studies for her meta-analyses based on predetermined criteria and ensures that the studies are indeed comparable and can be analyzed in the same meta-study. Then, for each of these studies, she calculates a ‘summary statistic’, a quantity that captures the effect of interest, like the difference between the averages of the control and test groups. All these summary statistics are then put together into one pooled measure. If the different studies do not share the same design, analysing the pooled data must take this into account. For example, if an average measure is calculated from the measure from the individual studies, the average will be ‘weighted’, so larger and probably more accurate studies contribute more to that average.
One of the most common ways of doing a meta-analysis is to figure out something called the ‘meta-analytic effect size’. ‘Effect size’ is a technical way of saying how big the difference between the two groups is, and in what direction that difference goes. If one were to statistically compare the height of Scandinavian men and Korean men, and see that Korean men are on average shorter, then that difference in height between the two groups is the effect size. This can either be presented as is, with the units in centimetres of height (in this example), or it can be ‘standardized’ and presented without any units through a mathematical formula. Cohen’s d, for instance, is one of the most commonly used standardized effect sizes.
The ideal effect size is not only detectable with a reasonable sample size but denotes a biologically meaningful difference. Indeed, the tinier your effect size, the larger the sample size required to detect it, which is one of the reasons a power analysis is recommended before carrying out an experiment. A power analysis simply seeks to ask “This is the effect size I care about, and that is the statistical power I care to have. How do I design my experiment and how many participants or subjects do I need to show this effect with those statistics?” A well-designed experiment might reveal that a drug truly reduces the size of tumours, but if twenty-thousand genetically-modified mice had to be sacrificed to show that about twenty-five of them had reduced tumours, the effect size is pointlessly small, few people will care and funding agencies will ask a lot of awkward questions.
Nevertheless, a small effect size is not necessarily meaningless. Meta-analyses show, for example, that programmes that work to improve self-esteem in school children tend to have a far higher effect-size than those that work to reduce violent behaviour. So, any new study that reports an effective school program needs to justify itself against the effect size usually seen for that type of program. Similarly, diabetes treatments have enormous effect sizes compared to treatments for either depression or schizophrenia. Context matters.
No philosopher’s stone
A meta-analysis cannot tell a reader what its composite studies don’t tell it. While they can reveal overall patterns and small but meaningful effects that cannot be seen in individual studies, the fact remains that they cannot transmute base bad research into sound conclusions on a gold standard. In other words, meta-analyses are prey to the ‘garbage in, garbage out’ principle.
It’s not just what goes into a meta-analysis that matters, but what doesn’t. Many scientists have, lost in a labyrinth of half-forgotten folders in their hard drives, at least some data from an experiment that was never published. They could be entire experiments, possibly very well-designed, or individual analyses that yielded uninteresting results. This is so common that the phenomenon has a name—the ‘file-drawer problem’—even if this does sound a little archaic. The implication of the forgotten file-drawer is clear—published data are much easier to include in a meta-analysis, and published studies are much more likely to have ‘significant’ results.
The signature of missing data can actually be detected by some clever tools, and one sophisticated method, fairly recent, is the p-curve analysis, which plays around with the p-values of studies. It is a sort of ‘key to the file drawer’ because it does not require those unpublished results to reveal that data have gone missing in action.
The p-value is essentially a probability measure. It tells you how likely your data are if you assume that your null-hypothesis is true, or that the drug, protocol or whatever intervention you are testing doesn’t have any effect. A p-value that is less than 0.05 is taken by convention as ‘significant’. The value is supposed to be low enough that the null-hypothesis is very unlikely, and the intervention has indeed worked. ‘0.05’ simply means that there is a chance of only 5 in 100 or 1 in 20 that the significant result is a false-positive, and the statistics picked up an effect where none existed.
If all that ever happened was publication bias, and scientists only published those experiments that turned up below 0.05 then 95% of all the work ever done would never see the light of day; not so much a ‘file-drawer’ as a ‘floor-to-ceiling’ file-cabinet! It is more common for scientists to ignore, not entire experiments, but only those analyses that yield non-significant results, and keep trying different statistical tests until they’ve got the p-value they want. This is the notorious and unethical practice of p-hacking, a much better shot at throwing up false-positive findings than running the same experiment a hundred times over.
The p-curve simply takes the significant p-values from many different studies, counts how many of those studies fall in the different parts of the 0 to 0.05 range and plots this as a frequency distribution or ‘curve’. Depending on what has happened, the curve takes on different shapes. When no data are missing and the effect is a true effect (the null-hypothesis is false), the curve is skewed so that most of the p-values are very small. When no data are missing but there is no true effect, all p-values are equally likely, and the curve is flat. When there are data missing through p-hacking or publication bias, the curve is skewed so most p-values, though smaller than 0.05, are still close to 0.05. This is because, when they p-hack, most scientists stop (mis)analyzing their data as soon as they cross that magical threshold of 0.05; rarely do they carry on until they’ve hit 0.01 or lower.
Figure 1: p-curves in different situations (figure from Simonsohn et al., 2014). The top row shows that when p-hacking has not occurred, the curve is right-skewed when the statistics have sufficient power to detect a true effect (B-D) and flat when there is no effect (A). The bottom row shows that when p-hacking has occurred, the curve is left-skewed when there is no effect (E), and the combination of a true effect, sufficient power and p-hacking will produce a left-skewed curve again (F-H)
Gate-keeping: What studies go into a meta-analysis?
Let us postulate that medical researcher wants to do a meta-analysis of twenty studies that look at the effect of exercise on heart health. Each of those studies might be small and not very conclusive, but the data can nevertheless be combined to produce a more reliable result. One of the first things the scientist would have to figure out is what sort of data is included in her meta-study—who are the patients in the smaller studies she can take data from. They could be people who have suffered heart-attacks, professional athletes, teenagers, people in their eighties, people who have had heart-transplants or those born with heart defects that were surgically repaired. A seventy-five-year-old who has chain-smoked her whole life is unlikely to enjoy the same benefits of exercise that an abstinent twenty-one year old would, so it’s very important that the meta-statistics adequately account for this.
Next, the scientist has to decide exactly what data she is looking at and make sure they are comparable. Most of the twenty studies in her analysis might have been ‘interventions’—putting carefully selected study participants on an exercise program they weren’t used to. Some might simply have been observational and connected the incidence of heart-disease with hours per week spent exercising in a random selection of people. These types of studies aren’t necessarily comparable, and the meta-analysis must either exclude them or figure out what measurement can be safely taken from all of them to put into the same statistical tests.
Then, a ‘summary statistic’ is calculated for all of the papers that go into the meta-analysis, and then all of these are put together into one pooled measure. Because the different studies are likely not designed the same way, analysing the pooled data has to take this into account in different ways. Further, the meta-analysis must also take into account whether all the composite studies are estimating exactly the same true quantity in the same way.
If meta-analyses can only be as good as the studies that go into them, p-curves are only the beginning. As the above example shows, every step of the way, there is room for misinterpretation, misrepresentation and miscalculation. The choice of which studies to take for a meta-analysis is absolutely critical because precious few researchers set up their experiments as ‘future components of a meta-analysis’. Studies can have vastly different methods—protocols, doses, control groups or experiment designs—and draw their data from vastly different participants or individuals, even if they are studying approximately the same thing.
There can be a strong subjective element when choosing which studies go into a meta-analysis and two meta-analyses selecting two different groups of papers that try to answer the same question could well wind up with completely opposite conclusions. Depending, essentially on who you ask, you could either conclude that an increase in the minimum wage decreases or increases unemployment and psychotherapy is effective or no better than a placebo.
Meta-analyses have proliferated in the last few years, and, like individual papers, can be plagued with flaws. John Ioannides, the man whose paper “Why most published research findings are false” has been credited as ‘making scientists question themselves’, estimates that 60% of all meta-analyses are flawed, misleading or redundant. This is through a combination of overlapping topics, conflicts of interest of the authors and funding agencies, analysis of completely outdated information and inappropriate statistics.
The jigsaw without a reference
Asking whether meta-analyses are ‘worth doing’ is like asking whether science is ‘worth doing’ because a lot of studies cannot be replicated. A well-done meta-analysis can reveal over-arching patterns that single studies cannot, narrow in on true effect sizes, and perversely, reveal the existence of bias, missing data and p-hacking. Meta-studies have had significant impact on the medicine is done. They guide the treatment of disease and even put the lie to claims accepted as common knowledge, such as artificial sweeteners causing cancer. They are even known to be life-saving. It took meta-analyses to reveal the large increase in mortality due to artificial blood products as opposed to normal donated blood, the likely presence of the ‘weekend effect’ in the hospital mortality rates, and the strong link between heart attacks and a diabetes medication from pharmaceutical giant GlaxoSmithKline; the last of these was even confirmed in an updated analysis a couple of years after the original paper. Meta-analyses have also completely rubbished the link between the MMR vaccine and autism, though, tragically, this has not convinced nearly as many people as it should have, and children continue to die from diseases we could have easily eradicated. Another meta-analysis from 1990, however, did save the lives of children: by putting together data from 12 studies it showed that giving steroids to mothers who were expected to deliver babies prematurely increased the survival rate of the babies, something that had been questionable until then because not all the available evidence seemed to support it. The critical figure from this study became the logo of the non-profit Cochrane Collaboration, founded in 1993 and aimed at producing reviews and meta-analysis to aid in evidence-based medicine..
Perhaps the problem is not so much the principle of combining multiple studies into an overall picture but the fact that it is done retrospectively. Lots of studies are done in their own merry ways, someone decides to do a meta-analysis to see the ‘state of the field’, puts together the studies as carefully as they can and tries to see the big picture. As has always been the case with science, though, there is no reference picture on the container of the puzzle—we can never know with complete certainty the real, objective truth. The best we can do is get better and better approximations of it.
Putting together studies in a meta-analysis to try to find a conclusive answer is the equivalent of gathering together puzzle pieces that you’ve judged to come from the same box, figuring out the right way to fit them together from among many options, and try to judge the final picture without any sort of reference picture because you simply don’t have the puzzle box.
One partial solution lies in cumulative meta-analyses, where, as studies are done, they are added to a sort of ‘rolling analysis’ that continually updates the overall understanding of the question. Crucially, this sort of cumulative analysis can be even more valuable than conventional meta-analysis because they can reveal potential disasters far earlier and even save countless lives. The study on artificial blood products referenced above, for example, also carried out a cumulative meta-analysis, adding studies to their analysis in chronological order to see the way their conclusions changed. The world would have known of the link between these products and high mortality as far back as 2000, eight years before the paper was published, if a cumulative analysis had been done from the beginning.
Ioannides points out that one day, prospective, instead of retrospective meta-analyses might become the norm, where planning and funding of smaller studies happen even before they are done with plans for future meta-analyses, standardized protocols and statistical methods in place. That day might never come; there will always be a place for exploratory studies that shoot in the dark so innovations can be discovered and new ground charted. In the meantime, as long as we continue doing meta-analyses to understand broader patterns, there is a lot to be said for careful criteria, looking for evidence of missing data, disclosing one’s methods, pre-registering the meta-analysis in a database just as one would an individual study, and rigorous and transparent statistics.
This article is the second part of the series ‘The How and the Why: Interpreting Scientific Studies’, brought to you by Research Matters. The series focuses on the method of scientific studies, including emphasising the importance of meta-analyses, the repercussions of the replication crisis and the inclusion of ethics in experimental biology. We hope this series will better enable our readers to understand and evaluate scientific research they are interested in and those that could impact their lives.